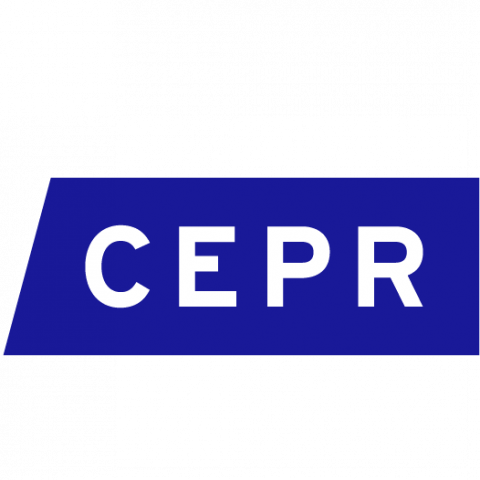
Low agricultural productivity in developing countries is not destiny, as there is remarkable potential for raising crop yields given their land quality
Editor's note: To know more about what countries in Africa can do to raise their crop yields, read our VoxDevLit on Agriculture in Africa.
In the public discourse, land quality and geography are often invoked as possible explanations for the low agricultural productivity problem plaguing developing countries. This notion stems from the fact that land quality and geographic attributes matter for farming productivity at the micro-level. But can geography account for the disparity in agricultural productivity between developed and developing countries, at the macro-level?
The importance of agricultural productivity
There are large differences in living standards across countries. Proxied by real gross domestic product per capita at common prices across countries, Ethiopia and Malawi had less than 3% of the GDP per capita of the US in 2014. A key determinant of these income differences is the fact that developed countries feature much higher aggregate productivity than less-developed countries (Klenow and Rodriguez-Clare 1997, Prescott 1998). In turn, a crucial determinant of aggregate productivity in less-developed countries is the prevalence of agriculture, and in particular low productivity in the agricultural sector (Gollin et al. 2002, Caselli 2005, Restuccia et al. 2008). Why is agricultural productivity so low in poor countries? The answer to this question has important implications for poverty reduction, welfare, structural transformation, and development.
Broadly speaking, there are two possible explanations for the agricultural productivity disparities across countries. First, there could be differences in geographic endowments across countries. According to this explanation, developing countries may have a natural disadvantage in agriculture due to low land quality, rugged geography, and arid lands. Agriculture takes place across space, using location-specific inputs, and as a result geography would matter more for agriculture than any other sector. Second, due to differences in institutions, constraints, frictions, or policies, countries may make different economic choices in agriculture, affecting the level of productivity (Restuccia et al. 2008, Adamopoulos 2011, Adamopoulos and Restuccia 2014, Gollin and Rogerson 2014, Tombe 2015, Donovan 2021, Sotelo 2020). Understanding which of these explanations is the main source of agricultural productivity differences across countries is essential and has dramatically different implications for policy.
Assessing the role of land quality
In our recent paper (Adamopoulos and Restuccia 2022), we quantify the role of geography in accounting for agricultural productivity differences across countries using high-resolution micro-geography data and a spatial accounting framework.
We use spatially gridded datasets on a rich set of land quality and geographic characteristics from the Food and Agricultural Organisation (FAO) ’s Global Agro-Ecological Zones (GAEZ) project (GAEZ 2000). The spatial unit of observation is a cell of roughly 10 by 10 kilometres in a grid covering the entire globe (about nine million cells). For each cell, GAEZ provides information on soil (depth, fertility, drainage, chemical composition), climate (temperature and moisture), and terrain (slope and elevation) – conditions that are relevant for agricultural production. Combining this data with state-of-the-art agronomic models that account for science-based biophysical growth requirements of crops, GAEZ also provides for each cell the potential yield of a crop, which measures the maximum attainable crop output possible, under assumptions about cultivation practices and water supply conditions.
Our baseline analysis assumes low-input cultivation practices (subsistence-based farming, labour-intensive techniques, and no application of fertilisers and pesticides) and rain-fed water supply, which are the conditions that require the least human intervention. In addition, we consider an alternative scenario of mixed-level cultivation practices and water supply conditions (rain-fed and irrigated). Our sample has very broad coverage including 18 crops for 162 countries.
We develop a spatial accounting framework that allows us to aggregate up from the cell-crop level to the country level where the aggregate yield (the real value of total output per hectare of land at common prices) can be written as a weighted average of the yields for every crop in each cell, weighted by the share of land in each crop and cell within the country. This decomposition is useful because it allows us to construct counterfactual aggregate yields depending on assumptions about the yield of each crop, the crops produced in each cell, and the location of production.
We perform a set of counterfactuals that incorporate the three potential channels of productivity variation connected with land quality: production potential, spatial potential, and total potential.
The production potential simply computes the aggregate potential yield using the potential yield for each crop in each cell, holding crop and location choices constant in each country.
The spatial potential is the production potential with location choices of crops that maximise agricultural output, holding constant the total amount of land for each crop in the country. This counterfactual combines production potential with a reallocation of crops across space to the most suitable locations.
The total potential is the spatial potential with crop choices that maximise agricultural output. This is the highest aggregate potential yield attainable in the country given geography conditions, combining production of crops at potential, reallocation across space to the most suitable locations, and reallocation of crop production to highest yields given geography.
Is land quality the culprit?
In our data set, we document that the aggregate actual yield differs substantially and systematically across countries. For instance, the average aggregate yield differs between the richest 10 % of countries and the poorest 10% of countries in the world by a factor of 3.1-fold. How much of this actual yield gap is due to geography differences?
The production potential counterfactual provides a first direct assessment to answer this question. Our constructed aggregate potential yield replaces the actual yields by crop and cell, with their corresponding potential yields under the low-input scenario and rainfed conditions. The results are striking. If the 10% richest and 10% poorest countries produced according to their potential, the yield gap would virtually disappear from 210% to 5%. In Figure 1, we plot the actual yield and the potential yield for each country in our sample against GDP per capita. While there is substantial variation in potential yields across countries - the ratio of the top to bottom deciles is six-fold - this variation is not systematically related to the level of development, as the actual yield is. For instance, the disparity in the production potential aggregate yield between Tanzania and Eritrea, two low-income countries, is roughly the same (around six-fold) as the disparity between Belgium and Austria, two high-income countries. In other words, the agricultural yield gap is not due to differences in geographic endowments across countries.
Figure 1: Aggregate actual and production-potential yield across countries—rainfed low input.
Figure 2 shows directly that the aggregate potential yield and actual yield are essentially uncorrelated. The main result is robust to alternative specifications such as using alternative weights to common international prices in aggregating across crops, or looking at individual crops such as wheat, rice, or maize.
Figure 2: Aggregate production-potential versus actual yield across countries—rainfed low input.
Our spatial potential counterfactual in addition reallocates production across space to maximise the aggregate yield. In this situation, we find that the aggregate yield disparity reverses to 0.94-fold, that is a difference of 6% in favour of poor countries. Changing the crop composition to maximise the aggregate yield has a larger impact, further reducing the rich/poor disparity to 0.78-fold, that is a productivity advantage for poor countries of 22%. Overall, exploiting the geographic potential in each country reduces the agricultural productivity gap from 210% to a 22% advantage for poor countries, reinforcing our conclusion that the agricultural productivity gap across countries is not due to geographic disadvantages in poor countries.
While the baseline analysis assumes potential yields under low-input and rainfed conditions, we also consider the main production potential counterfactual, under the alternative mixed-input scenario (Figure 3). We find that the conclusion about potential yields virtually eliminating the actual yield gap between the rich and poor countries is the same. However, under the mixed-input scenario, the potential yields are much higher than the actual yields in poor countries, with the gaps being three times higher than in rich countries. Consider for example, Germany and Mozambique. Germany is much more productive in actual yield than Mozambique. But the land quality of the two countries is similar (similar potential yield). Germany is producing almost at potential, while Mozambique is far from realising its potential. This implies that there is much more scope for poor countries in achieving higher potential yields.
Figure 3: Aggregate actual versus production-potential yields--mixed inputs.
Implications
We conclude that land quality and geography cannot justify the observed agricultural productivity gaps between developed and developing countries. This finding is encouraging because it means that there is scope for raising agricultural productivity in developing countries, which would have far-reaching implications for their structural transformation and development. Using a standard two-sector model, we show that a three-fold increase in agricultural total factor productivity due to increased potential yield, would reduce the share of employment in agriculture in poor countries from 70% to less than 15%, and reduce the income disparity with rich countries by more than half.
The key question then, is why aren’t poor countries more efficient in agriculture given their land quality? In terms of the research agenda on agricultural productivity, it is important to understand and quantify the constraints that prevent the adoption of modern technologies and the frictions that prevent markets from efficiently allocating resources in developing countries.
References
Adamopoulos, T (2011), “Transportation Costs, Agricultural Productivity, And Cross‐Country Income Differences”, International Economic Review 52(2): 489-521.
Adamopoulos, T and D Restuccia (2014), “The size distribution of farms and international productivity differences”, American Economic Review 104(6): 1667-1697.
Adamopoulos, T and D Restuccia (2022), “Geography and agricultural productivity: Cross-country evidence from micro plot-level data”, The Review of Economic Studies 89(4): 1629-1653.
Caselli, F (2005), “Accounting for cross-country income differences”, Handbook of Economic Growth 1: 679-741.
Donovan, K (2021), “The equilibrium impact of agricultural risk on intermediate inputs and aggregate productivity”, The Review of Economic Studies 88(5): 2275-2307.
GAEZ (2000), “Global Agro-ecological Zones” version 3.0. Food and Agricultural Organization (FAO) and International Institute for Applied Systems Analysis (IIASA).
Gollin, D, S Parente, and R Rogerson (2002), “The role of agriculture in development”, American Economic Review 92(2): 160-164.
Gollin, D and R Rogerson (2014), “Productivity, transport costs and subsistence agriculture”, Journal of Development Economics 107: 38-48.
Klenow, P J and A Rodriguez-Clare (1997), “The neoclassical revival in growth economics: Has it gone too far?”, NBER Macroeconomics Annual 12: 73-103.
Prescott, E C (1998), “Lawrence R. Klein lecture 1997: Needed: A theory of total factor productivity”, International Economic Review: 525-551.
Restuccia, D, D T Yang, and X Zhu (2008), “Agriculture and aggregate productivity: A quantitative cross-country analysis”, Journal of Monetary Economics 55(2): 234-250.
Sotelo, S (2020), “Domestic trade frictions and agriculture”, Journal of Political Economy 128(7): 2690-2738.
Tombe, T (2015), “The missing food problem: Trade, agriculture, and international productivity differences”, American Economic Journal: Macroeconomics 7(3): 226-58.