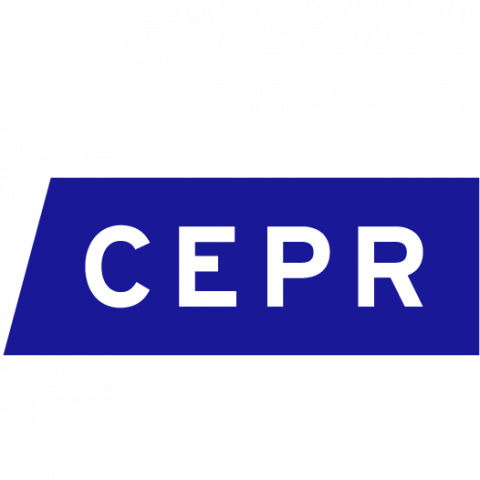
Energy-efficient technologies can mean more reliable electricity supply for everyone served by the same infrastructure whether or not they adopt the technologies
Energy-efficient technologies are frequently deployed via government programmes with the aim of inducing important electricity savings, reducing peak demand (Osborn and Kawann 2001; Gillingham, Newell, and Palmer 2006) and increasing reliability of electricity supply (World Bank 2006; Sarkar and Sadeque 2010). These goals of energy efficiency programmes are particularly crucial in low- and middle-income countries, where combined energy demand is expected to grow by 45% over the next two decades (IEA 2018).
Efficient lighting technologies, such as compact fluorescent lamps (CFLs) and light emitting diodes (LEDs), are a relatively accessible option for end-users and a popular choice of energy efficiency programmes. CFLs and LEDs are engineered to consume at least 75% less electricity per lumen relative to traditional incandescent bulbs. These technologies are also particularly pertinent for reducing peak demand in developing countries, where lighting comprises up to 86% of electricity consumption and consumption of lighting services typically occurs during peak times (Mills 2002).
Although programmes deploying efficient lighting are ubiquitous and ostensibly promising, there is little evidence of their impacts on household electricity consumption. Perhaps more notably, reliability effects of energy-efficient technologies and their implications for technology diffusion have received little attention. To affect electricity reliability, energy efficiency must reduce electricity consumption, in addition to also helping reduce congestion and overloads that occur when electrical grids are asked to deliver more power than system constraints allow — limited by either technical capacity or persistent power shortages (Lawton et al. 2003; Sullivan, Mercurio, and Schellenberg 2010; Singh and Singh 2010; Samad and Zhang,2016). Given the scale at which these effects typically occur, designing a study to identify such impacts on reliability is challenging.
Randomised distribution of energy-efficient lightbulbs
To estimate the impacts of energy-efficient lighting, we conducted a randomised experiment wherein we distributed CFLs in a district near the capital of the Kyrgyz Republic (Carranza and Meeks 2021). In our study setting, service provision is interconnected within a transformer; when a transformer has an outage, all households connected to the transformer are without electricity. In the years prior to our study, most local distribution transformers were strained, regularly operating at a load factor that substantially exceeded the maximum electricity load they could transfer at any given time (Amankulova 2006). As a result, transformer breakage, poor reliability, and unplanned outages were frequent (World Bank 2014), particularly during the winter when residential demand was high due to electric heating (World Bank 2017).
Through a randomised saturation design, we increased the energy efficiency of households. We employed the electric utility's data on residential users and the transformers through which they are served, and assigned treatment status in two stages:
- We randomised transformers to control, low or high CFL treatment saturations, with approximately 10 to 14% (15 to 18) of all households within low (high) treatment saturation transformers assigned to treatment.
- We randomised households individually to treatment and control groups, according to the saturation assigned to their transformer in the first stage.
Figure 1 shows a transformer in our research setting and depicts the random variation that results in both proximity to neighbouring treated households and the saturation intensity within a transformer. Households randomly assigned to treatment were offered the opportunity to purchase up to four 21 W CFLs (rated equivalent to 100 W incandescent bulbs) at a subsidised, randomly drawn price between 0 and .50 US$ per CFL. At baseline, households had 6.2 lightbulbs on average, of which only 0.2 were energy-efficient. Treated households received an average of 3.2 CFLs through this intervention.
Figure 1 Transformer in the research setting

Note: Photo of transformer that serves households at the research site in Kyrgyz Republic (photo credit: Robyn Meeks).
The impacts of energy-efficient lightbulbs
We observe three main results:
1. Households in the treatment arm with transformers and a higher CFL saturation report about two fewer days (in the prior month) without electricity due to unplanned outages. Basic differences in the distribution of outages by treatment status are shown in Figure 2 below. We consider these results as positive evidence that energy efficiency programmes can reduce peak loads to improve electricity reliability, provided they reach sufficient levels of technology saturation.
Figure 2 Stylised example of randomised saturation design

Note: A stylised example of the randomised saturation design (not to scale) to show how the randomisation resulted in different intensities of transformer treatment.
2. The impacts of CFL distribution on households' electricity consumption vary according to the CFL saturation within a transformer, as illustrated in Figure 3. At a lower saturation, CFLs reduce household electricity consumption and electricity savings are within the expected range from engineering calculation. However, at a higher saturation, CFLs allow them to increase their electricity consumption due to fewer outages.
Figure 3 Number of days without electricity, by transformer-level treatment status

Note: Graph uses household follow-up survey data from the question, "In the past month, how many days had your household been without electricity, due to problems with the electrical system in the village?" Control transformers have no households that received intervention CFLs. In treated transformers, some proportion received intervention CFLs.
3. Control households in treated transformers have significantly more CFLs than the “pure” control households in control transformers. Proximity matters in generating these adoption spillovers, which contribute to the electricity reliability impacts.
Figure 4 Household monthly electricity consumption effects, by transformer saturation


Note: CFLs were distributed, beginning in March 2013. The graph of the estimated impact was created by plotting the coefficients from regressing household electricity consumption (kWh) on household treatment status on a month-by-month basis, electricity consumption data provided by the electricity utility's billing. Additional regression details in paper.
Implications for energy efficiency policy
Through our CFL distribution, we show that adopting energy-efficient technologies can result in electricity reliability improvements. As electricity savings reduce the stress on the distribution infrastructure, sufficient saturation of efficient technologies can induce more reliable electricity supply for all end-users served by the same infrastructure, regardless of their own adoption of the technologies (Trifunovic et al. 2009). This improvement in reliability resulting from energy efficiency distribution is a classic example of a technological externality, through which an individual's returns to adoption increases discontinuously in the fraction of others adopting the technology (Foster and Rosenzweig 2010). Previous work has highlighted the importance such externalities in technology adoption, diffusion and subsidisation (Miguel and Kremer 2004; Cohen and Dupas 2010; Ashraf, Berry and Shapiro 2010).
Technology adoption spillovers such as improved electricity services provide a compelling rationale for mass deployment of energy-efficient technologies. These effects are important in the assessment of programme impacts and cost-effectiveness. In our study, the estimated benefits are more than double those based solely on the impacts on private electricity consumption. It is therefore, critical to determine the optimal scale of programmes delivering efficient technologies by calibrating the saturation needed to induce reliability effects. This requires considering the feasible impacts of the technology distributed, the contribution of the technology to on-peak electricity savings, the source of the constraint within the electricity system that typically causes outages, and the number of consumers served by the infrastructure.
Our findings are relevant to developing and developed contexts in which demand-side management is a potentially important tool in reducing electricity consumption. These include settings where infrastructure capacity is insufficient for current demand. That is where infrastructure will bind in the near future due to a rapidly increasing demand, or demand for electricity is congesting the infrastructure capacity. These constraints may not be related to distribution capacity, but to electricity generation. And they may be seasonal. For example, in countries that rely on hydropower, systems generation capacity may be insufficient during a dry season (Arias et al. 2020; DOE 2017). In developed countries, failures have also occurred during heat or cold waves when users run cooling or heating units (Aivalioti 2015; Dumas and Cunliff 2019). Also, where failure in distribution systems is less common, congestion within the electricity distribution network due to peak loads can impact utility prices (Lesieutre and Eto 2003) In those settings, energy efficiency may induce externalities in the form of lower electricity costs.
References
Aivalioti, S (2015), “Electricity Sector Adaptation to Heat Waves”, Columbia Law School, Sabin Center for Climate Change Law White Paper.
Amankulova, K (2006), “Transmission and Congestion Management in the Kyrgyz Republic”, National Agency for Anti-Monopoly Policy and Development of Competition (NAAPDC), Bishkek, Kyrgyzstan.
Arias, M F F, E Lee, A Livino, J Briscoe, and P Moorcroft (2020), “Impacts of Climate Change and Deforestation on Hydropower Planning in the Brazilian Amazon”, Nature Sustainability, 3: 430–436.
Ashraf, N, J Berry, and J Shapiro (2010), “Can Higher Prices Stimulate Product Use? Evidence from a Field Experiment in Zambia”, American Economic Review, 100(5): 2383–2413.
Carranza, E and R Meeks (2021), “Energy Efficiency and Electricity Reliability”, The Review of Economics and Statistics, forthcoming.
Cohen, J and P Dupas (2010), “Free Distribution or Cost-sharing? Evidence from a Randomized Malaria Prevention Experiment”, Quarterly Journal of Economics, 125(1): 1–45.
DOE (2017), “The Effects of Climate Change on Federal Hydropower: The Second Report to Congress”, U.S. Department of Energy.
Dumas, M, B Kc, and C Cunliff (2019), “Extreme Weather and Climate Vulnerabilities of the Electric Grid: A Summary of Environmental Sensitivity Quantification Methods”, U.S. Department of Energy, Office of Scientific and Technical Information.
Foster, A and M Rosenzweig (2010), “Microeconomics of Technology Adoption”, Annual Review of Economics, 2(1): 395–424.5
Gillingham, K, R Newell, and K Palmer (2006), “Energy Efficiency Policies: A Retrospective Examination”, Annual Review of Environment and Resources, 31(1): 161–192.
Lawton, L, M Sullivan, K V Liere, and A Katz (2003), “A Framework and Review of Customer Outage Costs: Integration and Analysis of Electric Utility Outage Cost Surveys Costs”, Lawrence Berkeley National Laboratory, University of California Berkeley, Berkeley, CA.
Lesieutre, B and J Eto (2003), “Electricity Transmission Congestion Costs: A Review of Recent Reports”, U.S. Department of Energy, Office of Scientific and Technical Information.
IEA (2018), “Perspectives for the energy transition? The role of energy efficiency”, Discussion papers, International Energy Agency.
Miguel, E and M Kremer (2004), “Worms: Identifying Impacts on Education and Health in the Presence of Treatment Externalities”, Econometrica, 72(1): 159–217.
Mills, E (2002), “The 230-billion Global Lighting Energy Bill”, Lawrence Berkeley National Laboratory, Berkeley, CA.
Osborn, J and C Kawann (2001), “Reliability of the U.S. Electricity System: Recent Trends and Current Issues”, Environmental Energy Technologies Division, Ernest Orlando Lawrence Berkeley National Laboratory, Berkeley, CA.
Samad, H and F Zhang (2016), “Benefits of Electrification and the Role of Reliability”, World Bank Policy Research Working Paper.
Sarkar, A and Z Sadeque (2010), “Bangladesh Set a World Record: 5 million CFLs in One Day”, The World Bank, Washington, DC.6
Singh, R and A Singh (2010), “Causes of Failure of Distribution Trans-formers in India”, International Conference on Environment and Electrical Engineering, Prague, Czech Republic.
Sullivan, M, M Mercurio, and J Schellenberg (2010), “Estimated Value of Service reliability for Electric Utility Customers in the United States”, Lawrence Berkeley National Laboratory, University of California Berkeley, Berkeley, CA.
Trifunovic, J, J Mikulovic, Z Djurisic, M Djuric, and M Kostics (2009), “Reductions in Electricity Consumption and Power Demand in Case of the Mass Use of Compact Fluorescent Lamps”, Energy, 34(9): 1355–1363.
World Bank (2006), “Improving Lives: Program on Renewable Energy and Energy Efficiency”, The World Bank Group, Washington, DC.
World Bank (2014), “Power Sector Policy Note for the Kyrgyz Republic”, The World Bank, ESMAP, Washington, DC.
World Bank (2017), “Analysis of the Kyrgyz Republic’s Energy Sector”, World Bank, Energy and Extractives Global Practice, Washington, DC.