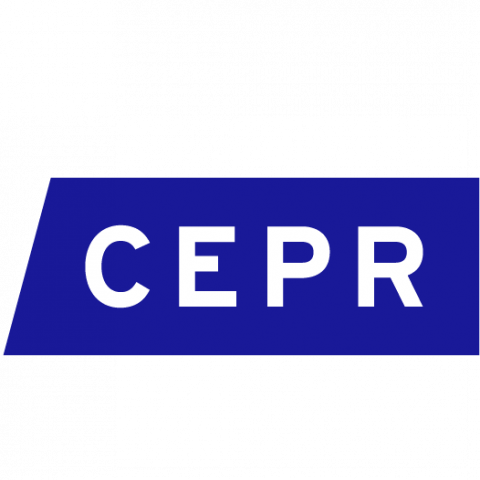
Big data with big theory reveal how restrictive regional economic policies can lead to lower national productivity and higher inequality
Within a given country, different regions interact with each other in capital, labour, and product markets, resulting in cross-regional flows of these factors and goods. Regions also differ from each other locally in many ways, including the specific financial obstacles faced by local residents (Paulson and Townsend 2004, Ahlin and Townsend 2007, Karaivonov and Townsend 2014). Can these regional differences be enough to generate flows of factor inputs that are consistent with the capital flows and labour migration seen in the available data? Furthermore, can these regional differences generate the observed and often quite uneven geographic concentration in economic activity that we see on the ground?
The study
In a recent study, we have answered these questions with resounding affirmatives (Moll et al. 2017), and our results have important implications for the debate on populism and nationalism. In the current economic climate there are pressures for regional isolation within countries (for example, within Spain) and across countries (as in the case of Brexit). Urban or industrialised areas, or entire countries, might contemplate restrictions on inter-regional or international labour migration with the belief that this might be helpful to local residents, in particular by raising local wages, or preventing them from falling. Likewise, rural areas and less developed countries may contemplate restrictions on outflows of capital to try to keep funds at home.
Through the lens of the calibrated model in our paper, we discover that the effect of these restrictive policies taken together would be severe and often result in unanticipated outcomes, even for target beneficiaries. Economic life in cities would suffer enormously, as would rural and national productivity, with a resulting overall increase in inequality.
Big data
In our paper, we use big data to uncover new findings and guide policy. Though big data is frequently thought of as the use of large administrative datasets, it can include other types of data, such as studies where there is both a complexity and variety of data that needs to be linked, connected, and correlated. This is the case with the Townsend Thai Project. It has monthly and annual data, in both villages and in towns/cities, with various instruments for households, heads of villages, soil/water conditions, local financial institutions, and joint liability groups.
Big theory
The term ‘big theory’ is used here as a counterweight. We use a theoretical model to organise data and to make predictions. It is a rather challenging model to solve, given the complexity that comes with the realism. But using this combination of big theory and big data yields those surprising implications regarding factor flows and geographic concentration.
The setting: Thailand
My long-term panel data collection project in Thailand, an emerging market country, provides a rich source of data for just this kind of effort (Townsend 2016). We selected provinces that varied in their levels of development, with two in the relatively poor agrarian Northeast, and two in the more developed Central region near Bangkok. We expand later with two additional provinces, in the North and in the South.
We field surveys on a monthly and annual basis in stratified random samples of rural villages and urban neighbourhoods that are representative of their provinces. We now have 20 years of rural annual and monthly data and more than ten years of urban annual data.
From these data, we are able to construct actual integrated financial accounts (income statements, balance sheets, cash flows) at both the household and the small and medium-sized enterprise (SME) levels. From the monthly data, we have village and region level income and product accounts, essentially the local GDP, as well as the capital flows (balance of payments surplus or deficit and flow of funds accounts).
The model
In theory and in practice, people in households make decisions about what they will do – work for themselves (run a business) or for someone else (wage work). In our model, as in the real world, people vary in latent talent for business following an exogenous stochastic process, for each over time, and they face various possible obstacles in financing and insurance.
Financial service providers compete to offer contracts to clients, provide credit and savings instruments, and risk-pooling instruments such as mutual funds. These financial intermediaries face economy-wide wage and interest rates, which in the model, have to have clear labour and capital markets in equilibrium. We are thus solving a rich contracting problem involving occupational choice and production decisions for households that differ in their wealth while respecting incentive and limited commitment constraints that are different in different regions.
Moral hazard and limited commitment
We found from earlier research using this micro data that there is a moral hazard problem for households and SMEs in the Central region and in urban areas, and a limited commitment, capital constraint problem in the Northeast region and in rural areas.
These findings are consistent across three diverse pieces of previously published research:
- occupation choice (Paulson et al. 2006);
- cross sectional and panel data on income, consumption and capital of entrepreneurs (Karaivanov and Townsend 2014); and
- loan repayment rates (Ahlin and Townsend 2007).
For example, if there is a limited commitment problem – i.e. when borrowers can walk away from loans – then collateral is needed, and thus the higher the wealth, the higher the debt. In contrast, in the moral hazard model, high debt is a burden on entrepreneurs with a perverse incentive to be less diligent, and so self-financing is a way to overcome the problem; thus, debt decreases with pre-determined wealth.
In the moral hazard financial regime, capital responds more quickly to productivity shocks than in the limited commitment regime, where there is large persistence. Limited commitment can be mitigated by official and unofficial penalties, with higher loan repayment and lower default rates. In our data, we see variation in those penalties and repayment rates across villages in the Northeast that are consistent with limited commitment being the key ingredient. All of these predictions across diverse theories are confirmed in our data with a consistent pattern when we stratify by regional industrialised versus agrarian and/or by urban-rural status.
Findings
In calibrating our model with the data, we find:
- Large capital flows: 23% of capital in industrialised areas is imported from rural, agrarian areas, which is 40% of the rural households’ wealth.
- Huge flows of labour in the same direction: 75% of labour in the urban areas comes from this migration, and rural areas lose 85%.
- Regional concentration: Urban/industrialised areas use 79% of the economy’s capital and 65% of its labour even though they only account for 30% of the population.
- Growth of net worth will be more concentrated in the Central region, and this is reflected in the data.
- Firm size distribution by capital predictions are also consistent with the data.
Test: Counterfactual policy experiment
A counterfactual policy experiment can often be quite illuminating. Having seen how well the model matched the actual data, we then explore the effects of hypothetical policies that would have the intent of ‘protecting’ regions from cross-regional flows of capital and labour – i.e. protecting the wages of workers in cities from labour inflow and protecting rural areas from capital outflow.
As an extreme case, we completely shut down resource flows and move to regional autarky (self-sufficiency). In this counterfactual, the positive consequences for households in rural and less developed areas are:
- increases in overall average consumption, income and wealth;
- increases in local labour and capital; and
- and decreases in local inequality.
Although on its own this might seem good for these households, there are large negative consequences:
- decreases in rural wages, which harms workers;
- decreases in interest rates, which harms savers; and
- and drops in local productivity.
In urban and industrialised areas, positive consequences are:
- some who remain as wage earners do benefit from higher wages; and
- some with savings benefit from higher interest rates.
The negative consequences, on the other hand, are:
- higher wages and higher costs of capital hurt households who would otherwise be running small businesses but cannot; and
- those higher costs also hurt those running larger businesses.
Overall, there are extreme drops in income, consumption and wealth in urban areas.
Taken together, the effects of these policies in both rural and urban areas lead to overall lower national productivity and higher inequality.
These counterfactual exercises showed us that, if we had instead imposed financial frictions without looking at the data, we would be getting different and misleading answers to our policy question. It is thus important to combine data and theory, not only from a methodological perspective but also from the crucial perspective of economic welfare.
Photo credit: Gerry Popplestone/flickr.
References
Ahlin, C and R M Townsend (2007), “Using Repayment Data to Test Across Models of Joint Liability Lending”, Economic Journal 117(517): F11-F51.
Karaivanov, A and R M Townsend (2014), “Dynamic Financial Constraints: Distinguishing Mechanism Design from Exogenously Incomplete Regimes”, Econometrica 82(3): 887–959.
Moll, B, R M Townsend and V Zhorin (2017), “Economic development, flow of funds, and the equilibrium interaction of financial frictions”, Proceedings of the National Academy of Sciences 114 (24): 6176-6184.
Paulson A L and R Townsend (2004) “Entrepreneurship and financial constraints in Thailand”, Journal of Corporate Finance 10(2): 229–262.
Paulson, A L, R M Townsend and A Karaivanov (2006), “Distinguishing Limited Liability from Moral Hazard in a Model of Entrepreneurship”, Journal of Political Economy 114 (1): 100-44.
Townsend, R M (2016), “Village and Larger Economies: The Theory and Measurement of the Townsend Thai Project”, Journal of Economic Perspectives 30 (4): 199-220.