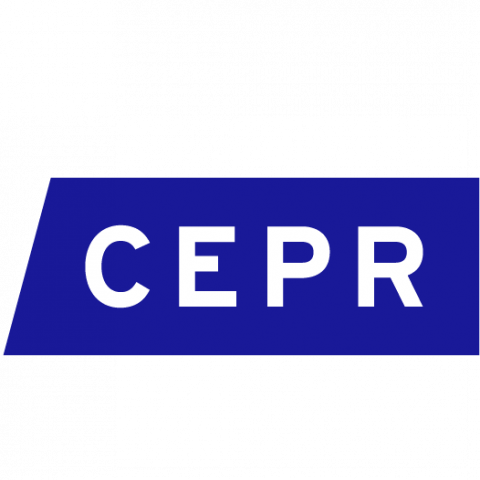
Implementation metrics explain much of the difference in effectiveness for a set of education programmes across studies and settings, playing a key role in generalisability
617 million young people worldwide are in school but unable to read fluently or perform simple numerical operations. These learning deficits are particularly acute in developing countries (UNESCO 2017, Angrist et al. 2021). Although many policies designed to address the learning crisis have yielded disappointing results, programmes which target educational instruction to a child’s learning level have improved learning in a variety of contexts (Banerjee et al. 2017, Muralidharan et al. 2019, Duflo et al. 2020, Angrist et al. 2023a). Randomised trials of targeted instruction approaches show consistently positive impacts in India, Kenya, and Ghana, receiving substantial attention in academic and policy circles (Global Education Evidence Advisory Panel 2020). However, while effects are consistently positive, they range from 0.07 to 0.78 standard deviations, varying by an order of magnitude. In this column, we present results from our recent paper studying this variation (Angrist and Meager 2023). We examine how generalisable effects might be, and uncover the factors behind the largest frontier effects in the literature.
We first assess the generalisability of targeted instruction by aggregating evidence across prior randomised trials. We then use the results to inform a new randomised trial, optimising delivery of a targeted instruction scale-up in Botswana. We consider data across eight study arms covering nearly 75,000 students across five contexts. We collect data on effect sizes as well as baseline learning, geographical context, sample size, year, and implementation delivery model (teachers or external volunteers). We also quantify programme implementation first using the notion of “take-up”, measured by attendance or presence of classroom materials. Second, we consider the “fidelity” of implementation, measured by adherence to core programme principles, such as whether instruction is targeted and students are grouped as expected. Targeted instruction is an ideal setting in which to study the different role of these two aspects of implementation, “take-up” and “fidelity”, as both vary widely across studies: take-up ranges from 8% to 90%, and fidelity from 23% to 83%.
Aggregating evidence across studies, accounting for implementation
We first aggregate the evidence using a frequentist approach, as shown in Figure 1, which aggregates effects across studies, and quantifies how much variation across studies is due to true treatment effect variation vs. statistical uncertainty. We find that interventions delivered by teachers have an average effect of 0.07 standard deviations (here we use Intention-to-Treat (ITT) effects). Teacher delivery effects also appear generalisable across studies in our data set (as signified by a low I-squared statistic), indicating that most of the variation in effects is due to sampling variation rather than true treatment effect heterogeneity. Second, we observe that volunteer delivery is on average three times more effective than teacher delivery with a 0.24 standard deviation effect. However, at first glance, the volunteer results are not as generalisable, and are highly heterogeneous (they have a high I-squared of 95.6%).
Figure 1: Meta-Analysis of Intention-to-Treat effects of targeted instruction programmes
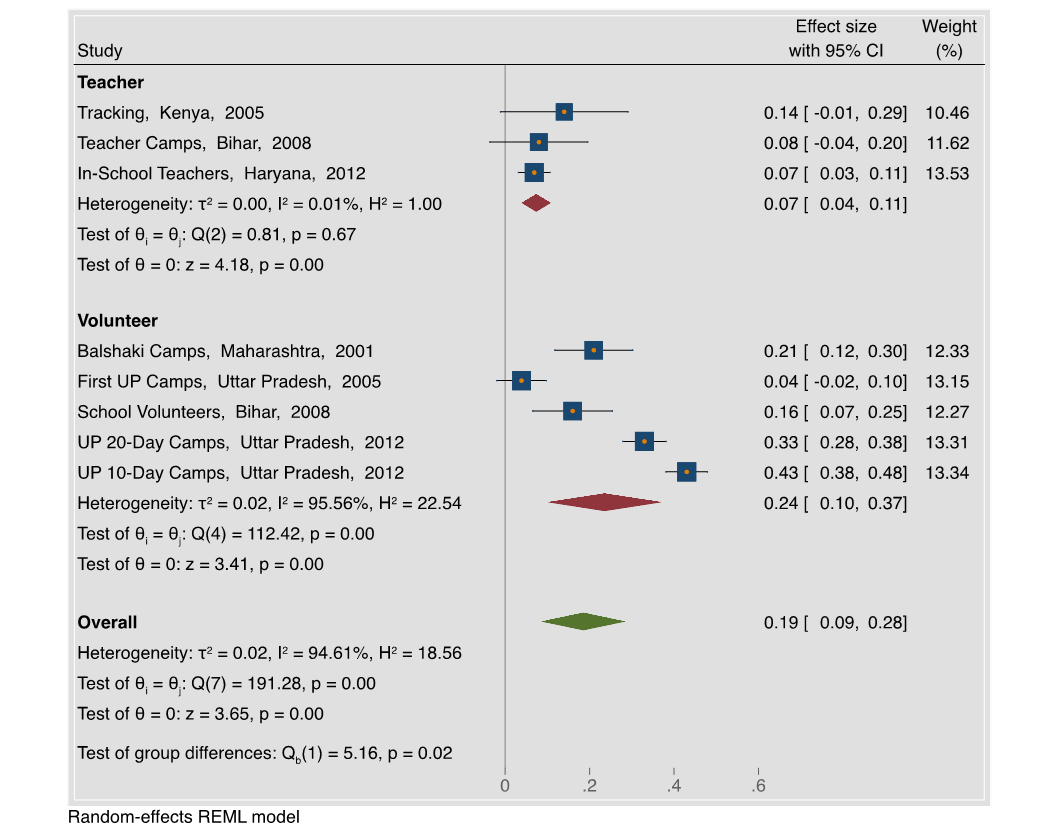
Next, we account for implementation. Using instrumental variables analysis, we estimate Treatment-on-Treated (TOT) effects across all studies – the effect on those who actually received treatment, not just those who were randomised to treatment. We then conduct a novel aggregation of Treatment-on-Treated (TOT) effects. This type of aggregation has to date rarely occurred in the evidence aggregation literature. Results are shown in Figure 2. We observe two trends: first, both teachers and volunteers are three times as effective conditional on implementation with 0.21 and 0.76 standard deviation average effects, respectively. These effects are large in a literature where average effectiveness is typically 0.1 standard deviations (Evans and Yuan 2020). Moreover, we now observe convergence among volunteer effects (with an I-squared of nearly zero). This reveals that much of the heterogeneity in the original volunteer estimates was due to variation in implementation.
Thus we find that implementation factors, including delivery model (teachers or volunteers) and the degree of implementation (ITT or TOT), explain most of the variation in the effects of targeted instruction programmes across settings. Accounting for implementation yields high generalisability across settings and explains the largest effects in the literature.
Figure 2: Meta-analysis of Treatment-on-Treated effects
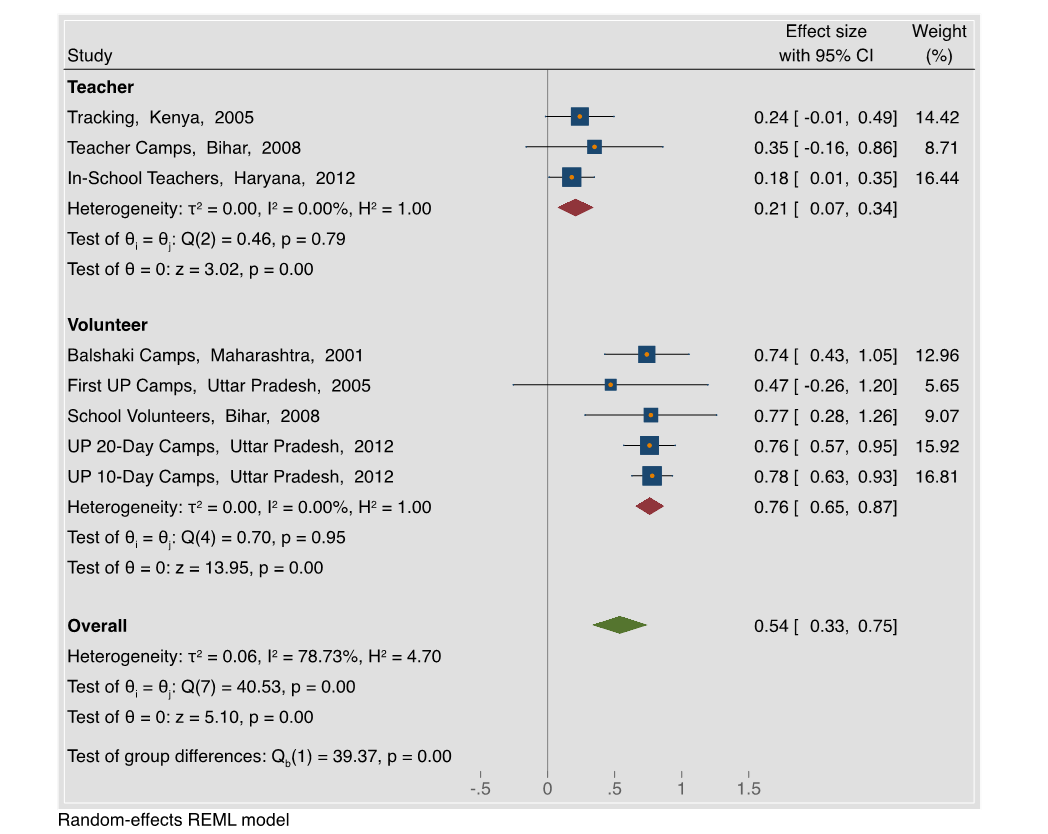
Incorporating implementation into evidence aggregation: a Bayesian model
Motivated by these results, we develop a new evidence aggregation model, which formalises the essential role of implementation information using a novel Bayesian hierarchical model. This model formally incorporates implementation into the evidence aggregation process, and enables us to account for uncertainty in implementation, just as there exists uncertainty in outcomes and treatment effects.
We further formalise the importance of implementation within this theoretical framework, showing that if implementation is not measured or accounted for, then treatment effects are not able to be accurately identified. For intuition, consider the case of null effects. Without accounting for implementation, it is impossible to differentiate if null treatment effects were due to an effective programme being poorly implemented or an ineffective programme that was implemented well. Both our results and this framework motivate greater reporting of implementation metrics. Such reporting has to date been rare and requires urgent attention: a review we conducted for this paper, showed that only 12.1% of studies currently report any implementation metric (Angrist and Meager 2023).
In our analysis using this Bayesian aggregation hierarchical model, we include two types of implementation metrics, which we refer to as m-factors: take-up as well as fidelity. Using our model, we show that targeted instruction can deliver 0.42 SD learning gains on average when taken up, and 0.85 SD gains when implemented with high fidelity, consistent with the upper range of effects found in prior studies.
Testing the causal impacts of implementation in the field
Given the importance of implementation identified in our evidence aggregation, a natural next question emerges: can implementation be rigorously studied and improved? We next empirically test whether there are concrete ways to increase take-up and fidelity of targeted instruction in the context of a scaling programme. We investigate approaches to increase the fidelity of targeted instruction in the case of Teaching at the Right Level (TaRL) in Botswana, where the government is actively scaling and testing the programme in partnership with Youth Impact, one of the largest NGOs in the country.
The standard implementation of Teaching at the Right Level in schools in Botswana involves testing and grouping students according to operational proficiency (e.g. addition, subtraction, etc). The new treatment randomly involved also sub grouping students according to their digit recognition level. For example, addition-level students who recognise 3 digits would be separated from addition-level students who recognise only 1 digit, with instruction further targeted to digit-recognition level. Results show that additional targeting improves learning by 0.21 standard deviations on average. These results confirm that the correlation between implementation and impact observed in the literature reflects a causal relationship – it is not merely the case that favourable settings yield both high implementation and large effects; rather, improving implementation directly improves programme results holding all else equal. These results also demonstrate that it is indeed possible to further optimise implementation in the context of a scaling programme.
Conclusion
Our analysis demonstrates the importance of quantifying programme implementation with as much care as we typically quantify programme effects. We find that implementation factors explain most of the variation in the effects of targeted instruction programmes across settings. This is welcome news: while many programmes work in one context, but don’t generalise well to the next (Vivalt 2020) or generalise only partially (Meager 2019), targeted instruction generalises very well when implementation is accounted for. Indeed, as shown in our study, external validity can be enhanced by simply reporting treatment-on-the-treated (TOT) effects in addition to the more typically reported intention-to-treat (ITT) effects. Reporting and aggregating TOT effects is likely to be important for many types of education interventions, and in other sectors too, such as health. A recent study estimating treatment-on-the-treated effects in the case of cancer screening trials also found this reconciled variation in effects (Angrist and Hull 2023). Further insight can be gained by using our new evidence aggregation model that formally accounts for uncertainty in implementation and also incorporates different notions of implementation.
We build on insights from our synthesis to guide a new trial optimising implementation in the context of a scaling programme. Through our trial in Botswana, we show that implementation can be changed in practice, identifying concrete mechanisms to achieve the largest effects in the literature. Given that targeted instruction yields up to 0.85 SD when delivered with high fidelity – 10-fold higher than the typical education intervention – future research on better implementing known productive interventions, such as targeted instruction, could have a higher return than discovery of new interventions.
Acknowledgments
We thank Abraham Raju for valuable research support for this article as well as Witold Wiecek and Veerangna Kohli for valuable research contributions to the paper. We are grateful to the American Economic Association, authors of the original research papers, and the Jameel Poverty Action Lab (J-PAL) which made all data easily accessible and available and advised on various components of the research. We thank Youth Impact who conducted randomised A/B tests on a scaling programme. The research and implementation were supported by the Center of Excellence for Development Impact and Learning (CEDIL) which is funded by the United Kingdom’s Foreign, Commonwealth & Development Office (FCDO) as well as the What Works Hub for Global Education, which is jointly funded by the United Kingdom’s Foreign, Commonwealth & Development Office (FCDO) and the Bill & Melinda Gates Foundation.
References
Angrist, N and R Meager (2023), "Implementation Matters: Generalizing Treatment Effects in Education." Working Paper. Available here.
Angrist, N, N Angrist, M Ainomugisha, S P Bathena, P Bergman, C Crossley, C Cullen, T Letsomo, M Matsheng, R Marlon Panti, S Sabarwal, and Sullivan (2023a), "Education children in emergencies: Global evidence from five randomized trials," VoxDev. Available here.
Angrist, N, S Djankov, P K Goldberg, and H A Patrinos (2021), "Measuring human capital using global learning data," Nature, 592(7854): 403-408.
Angrist, J and P Hull (2023), "IV Methods Reconcile Intention-to-Screen Effects Across Pragmatic Cancer Screening Trials," National Bureau of Economic Research, No. w31443.
Banerjee, A, R Banerji, J Berry, E Duflo, H Kannan, S Mukerji, M Shot-land, and M Walton (2017), "From proof of concept to scalable policies: challenges and solutions, with an application," Journal of Economic Perspectives, 31(4): 73-102.
Duflo, A, J Kiessel, and A Lucas (2020), "Experimental Evidence on Alternative Policies to Increase Learning at Scale," National Bureau of Economic Research, No. w27298.
Evans, D K and F Yuan (2020), "How big are effect sizes in international education studies?" Educational Evaluation and Policy Analysis.
Global Education Evidence Advisory Panel (2020), "Cost-Effective Approaches to Improve Global Learning: What Does Recent Evidence Tell Us Are 'Smart Buys' for Improving Learning in Low and Middle Income Countries?" Recommendations from the Global Education Evidence Advisory Panel, The World Bank.
Meager, R (2019), "Understanding the Average Impact of Microcredit Expansions: A Bayesian Hierarchical Analysis of Seven Randomized Experiments," American Economic Journal: Applied Economics, 11(1): 57-91.
Muralidharan, K, A Singh, and A J Ganimian (2019), "Disrupting Education? Experimental Evidence on Technology-Aided Instruction in India," American Economic Review, 109(4): 1426-60.
UNESCO (2017), "More Than One-Half of Children and Adolescents Are Not Learning Worldwide," UIS Fact Sheet No. 46.
Vivalt, E (2020), "How Much Can We Generalize from Impact Evaluations?" Journal of the European Economics Association, 18(6): 3045–3089.