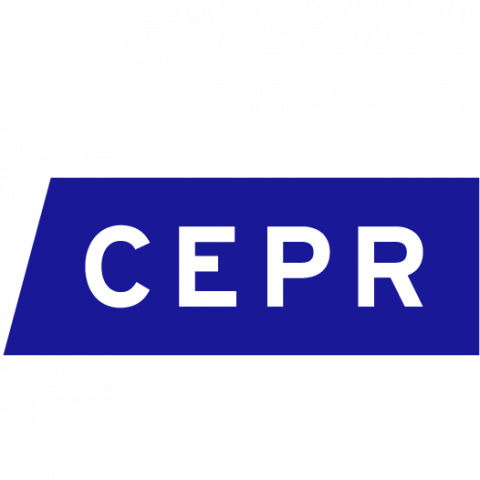
Satellite data are increasingly used to measure developing economies in areas including urban land use, agriculture and the environment
Researchers and policymakers in developing countries are often confronted with substantial data limitations. While a city planner in London can have detailed information about nearly any building's construction date and materials easily at hand, her counterpart in Nairobi is much less likely to have such information, especially for informal neighbourhoods. However, as Marx et al. (2016) show, publicly available data from remote sensing satellites can indicate roof material, as well as the age and/or maintenance of corrugated metal roofs. This allows them to measure housing investment and to show how it is affected by ethnic patronage in a Nairobi slum.
Economists increasingly use satellite data in their research, reflecting a broader trend towards "big data" that includes internet-sourced data (Edelman 2012), massive government administrative datasets (e.g. Chetty et al. 2013), and internal firm records (Einav and Levin 2014). It has been fuelled by related trends in technology like faster computers, in addition to decades of work by remote sensing scientists and geographers to build better satellites and interpretation methods. Satellite data are especially important for learning about the developing world, more so than these other forms of big data mentioned above. In a recent survey, we highlight exciting work in this area, and discuss the opportunities and challenges of satellite data (Donaldson and Storeygard 2016).
Merits of satellite data
While satellites are still expensive to build and launch, their price is decreasing quickly as more companies enter the market. Moreover, once they are in orbit, the additional cost of each photograph or other measurement they make is extremely low, allowing researchers to observe the same locations over time. By contrast, each additional round of a census or survey can be nearly as expensive as the first. Furthermore, most Earth observing satellites cover virtually all inhabited parts of the Earth, often in great detail, including war zones and places where conventional data are unavailable or untrusted such as North Korea (Lee 2018). Unlike administrative data, the timing and method of satellite data collection does not vary across province or even national borders.
Key application areas
- Lights as a proxy for economic growth: Economists have capitalised on each of the aforementioned features in an emerging literature in which satellite data on lights at night is used as a proxy for economic growth. Henderson et al. (2012) show that this proxy relationship is strong and consistent, and that it can be used to improve poor-quality estimates of GDP. Subsequent work has used lights as a proxy for GDP in a large sample of African cities (Storeygard 2016), border-crossing ethnic zones (Michalopoulos and Papaiannou 2014), and other regions where GDP is difficult to measure consistently.
- Environmental degradation: Another important area of application has been the way we monitor environmental degradation. Forest cover is easily seen from space and, increasingly, so is particulate pollution. In both cases, analogous ground-based measurements are often less consistent, and potentially subject to manipulation. Recent work has used satellite data to show how quickly and extensively Brazilian loggers react to changes in national forestry policy (Burgess et al. 2017), and to measure a substantial short-run impact of subways in decreasing air pollution in cities around the world (Gendron-Carrier et al. 2017).
- Urban land use: Satellites also have great potential for monitoring urban land use. Combining satellite and aerial data on building footprints and heights, Henderson et al. (2017) model the evolution of formal and informal neighbourhoods in Nairobi, quantifying built volume as well as the costs of delayed formalisation.
- Agricultural production: In general, there is a trade-off between the spatial resolution of images and their repeat frequency: for 5-metre resolution images that can discriminate between small farm plots, one typically has to wait long periods of time, whereas coarse 250-metre images may be available daily. However, high resolution satellites are proliferating, making this trade-off less severe. Weekly or even daily 5-metre (or finer) images within growing seasons will allow agronomists to more accurately measure and model crop growth (Lobell 2013).
Even in the absence of accurate production data as a proxy for agricultural conditions, economists are using climate model output that combines satellite-based information with weather station data (Auffhammer et al. 2013). These mixed products are better suited to distinguishing local variation in weather conditions than station data alone, especially in countries with only a handful of weather stations. For example, Costinot et al. (2016) use high-resolution agronomic estimates of crop suitability based in part on satellite-derived elevation data to model the macroeconomic impact of climate change on global agricultural markets.
Caveats and concluding remarks
In each of these areas, satellite data have allowed researchers to improve their answers to questions that had proven challenging before. One must caution that these data do not circumvent the importance of most kinds of traditional data on real quantities, prices, and attitudes from surveys and administrative sources. They only provide one view of the world (specifically, that from the heavens), and like many new data sources, they provide new scope for misinterpretation as well. However, their creative and careful use can fill important gaps in knowledge. With hundreds of new satellites now being launched every year and algorithms for interpreting them being advanced every day, the opportunities for improved research and policymaking are growing rapidly.
References
Auffhammer, M, S M Hsiang, W Schlenker and A Sobel (2013), “Using Weather Data and Climate Model Output in Economic Analyses of Climate Change", Review of Environmental Economics and Policy 7(2): 181–98.
Burgess, R, F Costa and B A Olken (2017), “The Power of the State: National Borders and the Deforestation of the Amazon”, Working Paper, London School of Economics.
Chetty, R, J N Friedman and E Saez (2013), "Using Differences in Knowledge across Neighbourhoods to Uncover the Impacts of the EITC on Earnings", American Economic Review 103(7): 2683-2721.
Costinot, A, D Donaldson and C Smith (2016), “Evolving Comparative Advantage and the Impact of Climate Change in Agricultural Markets: Evidence from 1.7 Million Fields around the World”, Journal of Political Economy 124(1): 205–248.
Donaldson, D and A Storeygard (2016), "The View from Above: Applications of Satellite Data in Economics", Journal of Economic Perspectives 30(4): 171-98.
Edelman, B (2012), "Using Internet Data for Economic Research", Journal of Economic Perspectives 26(2): 189-206
Einav, L and J Levin (2014), “Economics in the Age of Big Data”, Science 346(6210).
Gendron-Carrier, N, M Gonzalez-Navarro, S Polloni and M A Turner (2017), "Subways and Urban Air Pollution", NBER Working Paper no. w24183.
Henderson, J V, T Regan and A Venables (2017), "Building the City: Urban Transition and Institutional Frictions", CEPR Discussion Paper 11211.
Henderson, J V, A Storeygard and D N Weil (2012), "Measuring Economic Growth from Outer Space", American Economic Review 102(2): 994-1028.
Lee, Y S (2018), "International Isolation and Regional Inequality: Evidence from Sanctions on North Korea", Journal of Urban Economics 103: 34-51
Lobell, D B (2013), “The Use of Satellite Data for Crop Yield Gap Analysis”, Field Crops Research 143(1): 56–64
Marx, B, T M Stoker and T Suri (2016) “There is No Free House: Ethnic Patronage in a Kenyan Slum”, Working Paper.
Michalopoulos, S and E Papaioannou (2013), "Pre-colonial Ethnic Institutions and Contemporary African Development", Econometrica 81(1): 113-152
Storeygard A (2016), "Farther on Down the Road: Transport Costs, Trade, and Urban Growth", Review of Economic Studies 83(3): 1263-1295