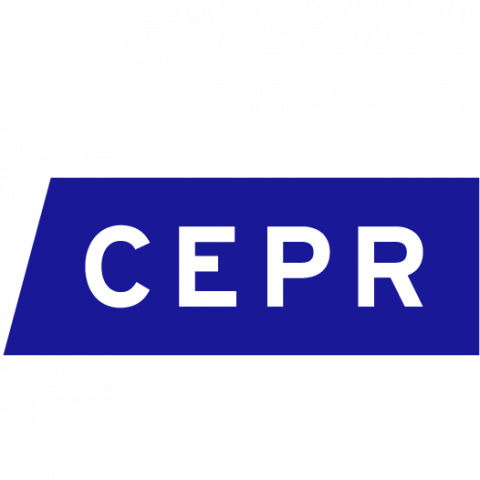
Commercialisation of microfinance institutions (MFIs) has long been a contentious issue.[1] For many people, the idea of using ‘market principles’ for the management of MFIs compromises the original idea of microfinance as a poverty reduction strategy. For others, it is the only sustainable path forward for the industry, with the often-heard ‘win-win’ proposition that by adopting commercial principles and practices MFIs can do more to reduce poverty.[2] One justification is that, by moving away from subsidy dependence, institutions will be able to grow beyond the limits of donor budgets, expanding their outreach to serve more people; but to be able to reach equity investors and disburse profits to shareholders, MFIs need to become fully regulated, commercial businesses. For those concerned about ‘mission drift’, the involvement of profit-driven shareholders limits social objectives.
Does commercialising microfinance undermine its social objectives?
A number of studies have explored the impact of MFI commercialisation. For example, de Quidt et al. (2018a) show that commercialisation leads to a decrease in joint-liability loans.[3] In this section, we begin by discussing the reality on the ground: how much of the microfinance sector continues to rely on subsidies?
The question of subsidies is critical when it comes to microfinance policy. In particular, how do we weigh the potential benefits of microcredit with its cost? Can we compare this cost-benefit ratio to other prominent interventions (such as cash transfers or vocational training programmes)? In this piece, we have primarily concerned ourselves with investigating the benefits of microcredit, which reflects the focus of most of the academic literature. Cull et al. (2018) address the other side of the ‘benefit-cost conversation’, using data from 1,335 MFIs between 2005 and 2009, jointly serving 80.1 million borrowers. They find that the costs of making small loans to poorer clients are high, with subsidies often necessary to deliver services when revenues do not cover costs. The authors use a method that accounts for the opportunity costs of all forms of subsidies (donated equity, borrowing at below market rates, and in-kind subsidies such as donated equipment, training, or labour). They find that the median institution receives five cents of subsidy per dollar lent and US$ 51 of subsidy per borrower (in PPP-adjusted terms). The relatively low levels of median subsidy suggest that even modest benefits of microcredit could yield impressive cost-benefit ratios. The distribution of subsidies is highly skewed, however, with an average subsidy of 13 cents per dollar lent and average subsidy per borrower of $248. The data also shows that subsidies per borrower are substantially higher for commercial microfinance banks and some non-bank financial institutions that make relatively large loans. MFIs that are organised as NGOs, catering to the poorest borrowers, generally relied less on subsidies. Overall, the results challenge the narrative that subsidies are initially helpful but will naturally disappear over time. The authors conclude that the long-standing debate about subsidies and commercialisation, which generally argues against the continued use of subsidies, appears to be out of alignment with realities on the ground. They suggest a transparent conversation about the uses and patterns of subsidies to understand how they can be used optimally. Another lesson we can draw is the importance of pursuing new ways to change the cost structure of MFIs, particularly with digital payments and innovations like mobile money, which have the potential to dramatically change business models (Suri 2023).
The crucial role of loan officers in microfinance
Next, we move to thinking more deeply about one of the main components of the cost of doing microfinance: staff. Because of the scarcity of readily available hard information on potential borrowers in poor countries, it is always challenging for loan officers to screen for high-quality clients.[4] As a result, it is critical to think about how to help loan officers select clients, disburse loans, and how they should be incentivised, with clear implications both for MFI repayment rates as well as the economic and social impacts for borrowers. Maitra et al. (2017) begin with the hypothesis that the failure of the traditional group-based microfinance product to increase borrower incomes (as discussed in Section 3A of this piece) was due to MFIs’ inability to successfully screen out unproductive borrowers.
The authors propose what they call ‘trader-agent intermediated lending’ (TRAIL), in which the bank delegates borrower selection to an agent chosen from informal traders and lenders in the community. Their hypothesis is that, if agents earn commission based on interest paid by recommended clients, this incentivises them to select borrowers who are less likely to default. Results suggest that the TRAIL model had large positive impacts: a 27% increase in the production of the leading cash crop and a 22% increase in farmer incomes. They also find that farmers selected by TRAIL agents were more ‘able’ than those self selected into the group-based model.
Identifying promising borrowers to help target microfinance
In a similar vein to Maitra et al. (2017), Hussam et al. (2022) explore the importance of selection and heterogeneous returns to capital, albeit not directly in a microcredit context. Specifically, they explore the impact of community screening for productive borrowers in Maharashtra, India. The authors ask local entrepreneurs in the community to rank their peers on metrics of business profitability and growth potential. There is an incentive for participants to rank their peers honestly in the form of rewards for accurate reports. To assess the validity of their reports, they randomly distributed cash grants to some entrepreneurs. The authors find that the information provided by community members is highly predictive of the marginal returns to capital: entrepreneurs ranked in the highest tercile earned returns that were three times the average return in the overall sample. Community ranking also outperformed a machine learning tool built using entrepreneur characteristics, implying that peer reports are predictive over and above observable traits.[5]
Similarly, Rigol and Roth (2021) conduct a novel field experiment with a large Chilean lender, to test whether organisational incentives for loan officers can hinder the graduation of promising borrowers. Specifically, the partner institution had recently introduced a separate graduation loan portfolio – implemented by different loan officers to the standard product, in a separate part of the organisation (with a separate management hierarchy). Under the status quo, loan officers were able to endorse lenders to move to the graduation portfolio. However, in doing so, loan officers risked losing compensation – because their remuneration depended upon the size and performance of their loan portfolio. Rigol and Roth study the sequential roll-out of two changes in loan officer compensation schemes.[6] The first change (‘mitigation’) counted graduated borrowers as if they remained part of the recommending loan officer’s portfolio, for the first six months. The second change (‘recognition’) provided rewards if graduated borrowers subsequently performed well (and retained the ‘mitigation’ reform). The authors find that the reforms caused an increase in graduation endorsements of about 5% for the ‘mitigation’ reform (1.1 additional endorsements) and then an additional 6% for the ‘recognition’ reform (making a combined increase of 2.1 additional endorsements: an increase of about 11%). Further, the authors show that loan officer endorsements are predictive of repayment behaviour (both in the standard joint-liability portfolio and in the graduation portfolio) – and that this result holds even after controlling for a vector of observable characteristics. The authors estimate that the combined effect of the ‘mitigation’ and ‘recognition’ schemes was to increase by 34% the net present value of graduation loans to endorsed borrowers – implying that strategic behaviour by loan officers is an important impediment to borrower graduation.
In recent related work, Bryan et al. (2024) run a novel experiment in Egypt, in which a large lender selected a sample of client firms that it believed might benefit from a larger loan; among this group, a control group had their loan size doubled, whereas a treatment group had their loan size quadrupled (with the treatment group offered, on average, 50% longer to repay). In comparing these groups, the authors find “mostly null average impacts of the larger loans, but important heterogeneity in treatment effects, along with strong evidence of misallocation”. The authors only discover this heterogeneity by including a combination of clients’ psychometric and cognitive data (that is, more standard data about business performance or client demographics does not suffice); this suggests that such psychometric data may be particularly important for lenders seeking to identify high-potential clients.
The findings of this set of papers (that is, Maitra et al. 2017, Hussam et al. 2022, Roth and Rigol 2021, Bryan et al. 2024) contrast with those of Vera-Cossio (2022), who finds that, in Thailand, community-allocated credit does not appear to flow to those who are needier, those who have higher total factor productivity (TFP), or those who appear to be most credit-worthy. Instead, credit appears to flow to households who are connected to members of the village council. A possible explanation for the difference between the West Bengal and Maharashtra findings of relatively efficient targeting and the Thai finding of elite capture is that in the Thai context there is little or no incentive for those allocating capital to ensure it flows to those with high returns. In a related paper, Maitra et al. (2024) show that allowing politicians rather than traders to allocate credit harms efficiency, partly due to changes in selection and partly due to changes in assistance given to borrowers by the intermediaries. Exploring ways to feasibly align lender incentives with the goals of efficiency and equity is an important area for further research.[7]
Earlier in this piece, we discussed the theory and evidence for how the group-based lending model (including features that leverage social capital over and above joint liability, such as group meetings and public repayment) can theoretically lead to positive selection. ‘Positive’ was generally taken to mean ‘low risk of default’, and – regardless of the muted impacts of microcredit on downstream borrower outcomes – there is no doubt that the microcredit business model has been tremendously successful at getting loans to the poorest borrowers and maintaining high repayment rates. However, given the findings in the literature that we have discussed in this piece as well as the debates around the economic impacts of microcredit for borrowers, these last two papers contribute to an exciting new strand of the literature that explores selection based on borrower productivity and thinks explicitly about microenterprise performance (potentially opening up new areas of microfinance product design, tailored at high-potential microentrepreneurs). In a sense, it brings us back to the apparent puzzle: why do we see high returns to microenterprises from capital grant studies, but not from microcredit impact evaluations? There are many possible explanations for this, and we have touched on the possible impact of relaxing contractual structures, but these last two papers open up an interesting frontier of thinking about selection and borrower productivity. More generally, understanding loan officer incentives and their interaction with borrowers is particularly important in a post-COVID-19 world where we may see large-scale defaults to microloans (after the expiry of widespread debt moratoria). In such an environment, understanding the incentives for loan officers is of critical importance for the welfare of the poorest borrowers (Malik et al. 2020).
References
Armendáriz, B and J Morduch (2010), The Economics of Microfinance, 2nd Edition, Vol. 1, Cambridge, MA: MIT Press.
Athey, S, D Karlan, E Palikot and Y Yuan (2023), “Smiles in Profiles: Improving Fairness and Efficiency Using Estimates of User Preferences in Online Marketplaces”, NBER Working Paper No. 30633.
Banerjee, A, E Breza, E Duflo and C Kinnan (2019), “Can Microfinance Unlock a Poverty Trap for Some Entrepreneurs?”, National Bureau of Economic Research Working Paper No. 26346.
Baraton, P and F Léon (2021), “Do Banks and Microfinance Institutions Compete? Microevidence from Madagascar”, Economic Development and Cultural Change 69(3).
Beaman L, D Karlan, B Thuysbaert and C Udry (2023), “Selection into Credit Markets: Evidence from Agriculture in Mali”, Econometrica 91(5): 1595 - 1627.
Bryan, G, D Karlan and A Osman (2024), “Big Loans to Small Businesses: Predicting Winners and Losers in an Entrepreneurial Lending Experiment.” American Economic Review 114(9): 2825–60.
Cull, R, A Demirgüç-Kunt and J Morduch (2018), “The Microfinance Business Model: Enduring Subsidy and Modest Profit.” The World Bank Economic Review 32(2): 221–244.
de Quidt, J, T Fetzer and M Ghatak (2018a), “Commercialization and the decline of joint liability microcredit.” Journal of Development Economics 134: 209–225.
De Quidt, J, T Fetzer and M Ghatak (2018b), “Market Structure and Borrower Welfare in Microfinance”, The Economic Journal 128(610): 1019-1046.
Hussam, R, N Rigol and B N Roth (2022), “Targeting High Ability Entrepreneurs Using Community Information: Mechanism Design in the Field.” American Economic Review 112(3): 861–98.
Karlan, D, A Osman and J Zinman (2023), “Dangers of a Double-Bottom Line: A Poverty Targeting Experiment Misses Both Targets”, Journal of Economics & Management Strategy 32(3): 510-522.
Macchi, E (2023), “Worth Your Weight: Experimental Evidence on the Benefits of Obesity in Low-income Countries”, American Economic Review 113(9): 2287-2322.
Maitra, P, S Mitra, D Mookherjee, A Motta and S Visaria (2017), “Financing smallholder agriculture: An experiment with agent-intermediated microloans in India.” Journal of Development Economics 127: 306–337.
Maitra, P, S Mitra, D Mookherjee and S Visaria (2024), “Decentralized Targeting of Agricultural Credit Programs: Private versus Political Intermediaries.” Journal of the European Economic Association 22(6): 2648–2699.
Malik, K, M Meki, J Morduch, T Ogden, S Quin and F Said (2020), “COVID-19 and the Future of Microfinance: Evidence and Insights from Pakistan.” Oxford Review of Economic Policy 36(Supplement 1): S138–S168.
McIntosh, C and B Wydick (2005), “Competition and microfinance”, Journal of Development Economics 78(2): 271-298.
Rigol, N and B N Roth (2021), "Loan Officers Impede Graduation from Microfinance: Strategic Disclosure in a Large Microfinance Institution." Working Paper.
Suri, T, J Aker, C Batista, M Callen, T Ghani, W Jack, L Klapper, E Riley, S Schaner, and S Sukhtankar (2023), “Mobile Money.” VoxDevLit 2(2).
Vera-Cossio, D A (2022), “Targeting Credit through Community Members.” Journal of the European Economic Association 20(2): 778–821.
Contact VoxDev
If you have questions, feedback, or would like more information about this article, please feel free to reach out to the VoxDev team. We’re here to help with any inquiries and to provide further insights on our research and content.