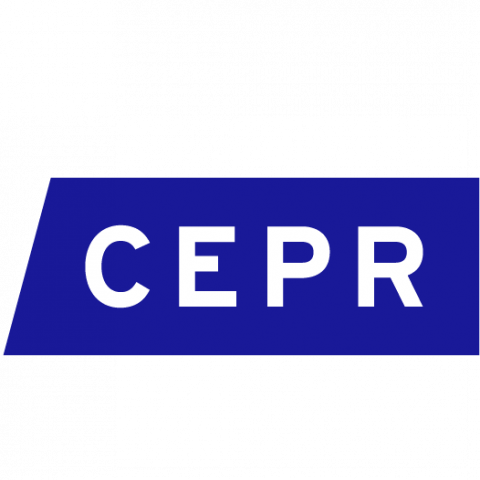
With more inputs, improved crop varieties can yield more. But do Ethiopian maize farmers know enough about their seeds to optimise fertiliser use?
Uncertainty about seed type and quality may lead to suboptimal fertiliser use
The Green Revolution was sparked by innovations in plant breeding and fuelled by complementarities between improved seeds and other inputs. However, the potential yield gains of better crop germplasm will not necessarily be realised unless farmers are able to optimise their input applications to the varieties that they are growing (Foster and Rosenzweig 1995). Hybrid maize, for example, yields much more than traditional varieties when optimally fertilised, but deviations from the optimum can rapidly reduce the economic returns (Duflo et al. 2008).
In the presence of such complementarities, farmers must know what they have sown in order to know how to adjust production inputs and practices. Uncertainty about seed type and quality can lead to costly suboptimal fertiliser use and slow learning about profitable agricultural technologies (Bold et al. 2017). At the national level, such on-farm misallocation could cause large input inefficiencies, with implications for seed system and input supply chain policies.
In our research (Bohr et al. 2024), we use novel nationally representative data from Ethiopia to compare farmer-reported seed types to the true genetic identity of their seed, as revealed by DNA fingerprinting (Kosmowski et al. 2020). We show that many farmers appear to be poorly informed about the genetic type of their seeds and that they apply inputs (in this case, chemical fertilisers) consistent with their beliefs rather than with the true genetic characteristics of their seeds. We find that about one-third of Ethiopia’s maize plots are managed by farmers who misidentify their seed type (where we consider two broad types: improved and traditional). We go on to show that this potentially leads to substantial aggregate effects on the allocation of fertiliser across maize farms in Ethiopia. These findings contribute to a broader literature on the challenges of technological progress and improving agricultural productivity in Africa (Suri et al. 2024).
Examining farmer beliefs and fertiliser choice in Ethiopia
Our choice of country and crop is motivated by several factors beyond the availability of genotyping data. First, improved maize seeds and chemical fertilisers have diffused widely across Ethiopia since the 1990s in response to government programmes and extension encouragement (Spielman et al. 2011, Abate et al. 2015, Kosmowski et al. 2020). Official recommendations advise farmers to use nitrogen fertilisers and recommend more nitrogen for hybrid (improved) maize than for non-hybrid maize (Abate et al. 2015).
Second, the Ethiopian context is also characterised by limited seed system regulation as well as widespread practices of informal farmer seed exchange and seed saving. This introduces substantial on-farm uncertainty about the genetic makeup of the seeds farmers sow and can render farmers’ survey-based reports of growing improved maize misleading.
Directly sampling maize at harvest and genotyping these kernels reliably (and ever more cost-effectively) reveals the true genetic identity of the seeds sown on a given plot (Stevenson et al. 2018, Beegle et al. 2012). Such genotyping has featured in a number of recent studies (Wineman et al. 2020, Maredia et al. 2016, Floro et al. 2018, Yirga et al. 2016). Our analysis assumes (consistent with the findings of Wossen et al. (2022)) that seed misclassification is due to misperception (i.e. false beliefs) rather than intentional misreporting.
Our paper builds on a pair of ‘double blind’ studies in Tanzania (Bulte et al. 2014, 2023) that experimentally introduce uncertain seed beliefs and observe how farmers’ behaviours change in response. Our context does not provide experimental variation; instead, we leverage the natural prevalence of false seed beliefs among farmers. Although our study lacks the causal clarity of an experimental intervention, we benefit from being able to draw on the variation in beliefs across a nationally representative sample of smallholder farmers. By observing farmers’ beliefs directly and studying their on-farm decisions, we can be confident in the external validity of our study.
There are potentially large costs at the farm and national level as many Ethiopian maize farmers hold false beliefs about the types of seeds they have sown
We find that nearly one-third of Ethiopia’s maize plots are managed by farmers who hold false beliefs about the types of seeds that they are growing. About half of these incorrect beliefs are that they are growing improved seeds; the other half appear to be growing improved seeds without being aware of it. This degree of seed misidentification is consistent with other emerging evidence (Wineman et al. 2020). The potential costs imposed by these false beliefs are evidenced in cumulative distributions of nitrogen applied by farmers (Figure 1), which suggest that farmer seed beliefs drive nitrogen applications much more strongly than the actual DNA type of the seed they sowed. Ethiopian farmers appear to believe (correctly) that improved maize seeds and nitrogen are complements.
Figure 1: Cumulative distribution of purchased fertiliser measured in nitrogen equivalents

Note: Cumulative distribution of purchased fertiliser measured in nitrogen equivalents by (a) DNA type at 95% purity threshold and (b) self-reported seed belief. Official nitrogen recommendations range from 110-130kg/ha (higher for hybrid (improved) maize) (Abate et al. 2015).
To understand how farmers’ beliefs about their seeds shape their nitrogen use, we classify farmers according to two binary variables. The first indicates whether the farmer reports having planted improved or traditional seeds, based on the post-planting round of the survey. The second indicates whether the genetic fingerprinting test for the post-harvest sample revealed that it is improved. We then estimate a model of nitrogen use that includes an increasingly rich set of controls, including a set derived from post double selection LASSO, to identify the impact of seed beliefs on input allocations.
As shown in Figure 2, which is based on our main results, farmers who believe they are growing improved maize apply comparable levels of nitrogen whether or not a plot is actually sown with improved or traditional seeds. These differences in input use are economically important: compared with “true negative” farmers growing the same traditional seeds, those with “false positive” beliefs (i.e. their seeds are traditional but the farmers believe they are improved) apply far more nitrogen fertiliser and thereby add an average of $84 or more to their per hectare input costs. This is the cost equivalent of paying an extra 20 days of wages in a growing season.
This misallocation can similarly be seen from the false negative perspective: farmers who falsely believe that they are growing traditional seeds use half or less the level of fertiliser chosen by farmers who correctly understand that their seeds are improved.
Figure 2: Estimated fertiliser application by farmer type

Note: The symbols indicate the point estimates for fertiliser application for four different seed belief types, and the vertical line is the 95% confidence interval.
Lastly, we leverage the nationally representative nature of our data to scale these on-farm results to national level fertiliser overuse and underuse. These results, shown in Figure 3, suggest that maize plots managed by farmers with false beliefs lead to substantial potential inefficiencies in the aggregate. Relative to their true belief counterparts, we project that farmers with false positive beliefs collectively overuse nitrogen by over 20,000 tons (14%), and false negative farmers underuse nitrogen by over 9,000 tons (7%).
Figure 3: Estimated national-level nitrogen use under observed and under correct beliefs
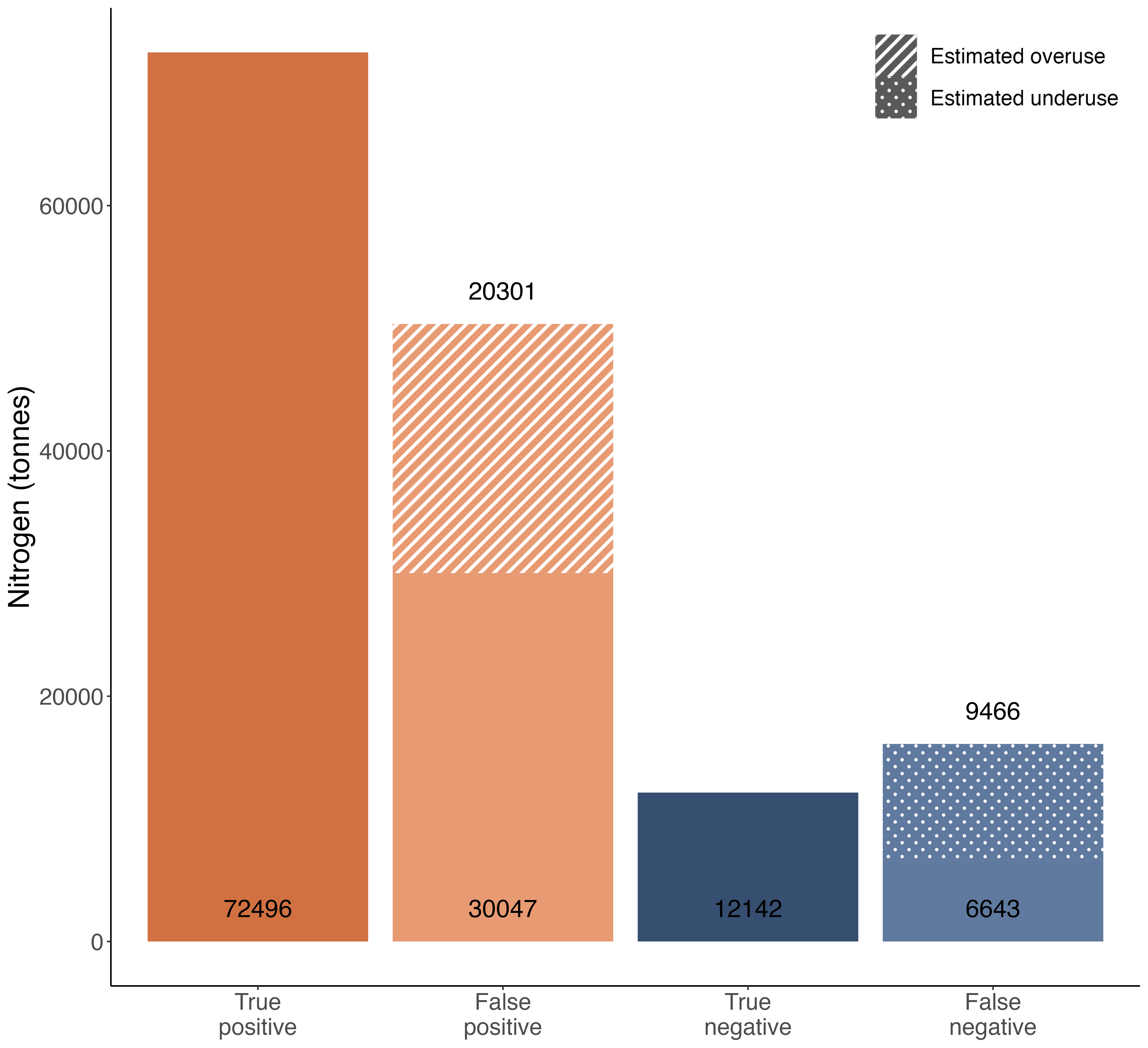
Note: “Overuse” and “underuse” are calculated with relevance to the application rates for farmers holding accurate beliefs about the genetic type of their varieties. Estimates are derived from plot-level calculations that adjust for plot, farm, household, and community covariates and location characteristics and that re-weight to all smallholder maize producers in Ethiopia. We note that farmers with accurate beliefs typically apply lower levels of fertiliser than recommended by government; our data do not allow us to assess the “true” optimal levels of nitrogen application.
Policy implications for agricultural programmes and research in sub-Saharan Africa
Our findings emphasise the importance of a deeper conversation about the diffusion of newly developed seed varieties and the driving forces of farmer misinformation. Combined with other emerging evidence, these findings raise the concern that such misinformation may be a prevalent problem elsewhere in sub-Saharan Africa.
Given how these small-scale inefficiencies aggregate to considerable input misallocation at the national and regional levels, understanding the factors contributing to accurate seed identification is a central issue for improving agricultural productivity. Only with improved measurement have we come to appreciate this potentially important source of on-farm inefficiency.
An additional lesson for development economists is that farmer self-reports as to seed type should be used with some caution. Calculations of the returns to the technology – or other micro analyses using ‘improved seed’ as either a dependent or independent variable – are likely to be flawed. It is also not clear that the errors in self-reports can be treated as classical measurement error; as we show in the paper, there are some systematic patterns in farmers’ erroneous beliefs. As other studies have previously shown, errors are sufficiently widespread to create consequential biases in results, and researchers should take account of this in study design and analysis.
References
Abate, T, B Shiferaw, A Menkir, D Wegary, Y Kebede, K Tesfaye, M Kassie, G Bogale, B Tadesse, and T Keno (2015), “Factors that transformed maize productivity in Ethiopia,” Food Security, 7: 965-981.
Beegle, K, C Carletto, and K Himelein (2012), “Reliability of recall in agricultural data,” Journal of Development Economics, 98(1): 34-41.
Bohr, N, T Deisemann, D Gollin, F Kosmowski, and T J Lybbert (2024), “The Seeds of Misallocation: Fertilizer Use and Maize Varietal Misidentification in Ethiopia,” Journal of Development Economics, 171: 103349. https://doi.org/10.1016/j.jdeveco.2024.103349.
Bold, T, K C Kaizzi, J Svensson, and D Yanagizawa-Drott (2017), “Lemon technologies and adoption: Measurement, theory and evidence from agricultural markets in Uganda,” The Quarterly Journal of Economics, 132(3): 1055-1100.
Bulte, E, G Beekman, S Di Falco, J Hella, and P Lei (2014), “Behavioral responses and the impact of new agricultural technologies: Evidence from a double‐blind field experiment in Tanzania,” American Journal of Agricultural Economics, 96(3): 813-830.
Bulte, E, S Di Falco, M Kassie, and X Vollenweider (2023), “Low-quality seeds, labor supply and economic returns: Experimental evidence from Tanzania,” Review of Economics and Statistics: 1-33.
Duflo, E, M Kremer, and J Robinson (2008), “How high are rates of return to fertilizer? Evidence from field experiments in Kenya,” American Economic Review, 98(2): 482-488.
Floro IV, V O, R A Labarta, L A Becerra López‐Lavalle, J M Martinez, and T M Ovalle (2018), “Household determinants of the adoption of improved cassava varieties using DNA fingerprinting to identify varieties in farmer fields: A case study in Colombia,” Journal of Agricultural Economics, 69(2): 518-536.
Foster, A D, and M R Rosenzweig (1995), “Learning by doing and learning from others: Human capital and technical change in agriculture,” Journal of Political Economy, 103(6): 1176-1209.
Kosmowski, F, S Alemu, P Mallia, J Stevenson, and K Macours (2020), “Shining a brighter light: Comprehensive evidence on adoption and diffusion of CGIAR-related innovations in Ethiopia,” SPIA Synthesis Report.
Maredia, M K, B A Reyes, J Manu-Aduening, A Dankyi, P Hamazakaza, K Muimui, I Rabbi, P Kulakow, E Parkes, T Abdoulaye, and E Katungi (2016), “Testing alternative methods of varietal identification using DNA fingerprinting: Results of pilot studies in Ghana and Zambia,” Working paper.
Spielman, D J, D Kelemwork, and D Alemu (2011), “Seed, fertilizer, and agricultural extension in Ethiopia,” Food and Agriculture in Ethiopia: Progress and Policy Challenges, 74: 84.
Stevenson, J, K Macours, and D Gollin (2018), “The rigor revolution in impact assessment: Implications for CGIAR.”
Suri, T, C Udry, J C Aker, C B Barrett, L Falcao Bergquist, M Carter, L Casaburi, R Darko Osei, D Gollin, V Hoffmann, T Jayne, N Karachiwalla, H Kazianga, J Magruder, H Michelson, M Startz, and E Tjernstrom (2024), “Agricultural Technology in Africa,” VoxDevLit, 5.2.
Wineman, A, T Njagi, C L Anderson, T W Reynolds, D Y Alia, P Wainaina, E Njue, P Biscaye, and M W Ayieko (2020), “A case of mistaken identity? Measuring rates of improved seed adoption in Tanzania using DNA fingerprinting,” Journal of Agricultural Economics, 71(3): 719-741.
Wossen, T, K A Abay, and T Abdoulaye (2022), “Misperceiving and misreporting input quality: Implications for input use and productivity,” Journal of Development Economics, 157: 102869.
Yirga, C, K Negisho, L Oruko, D Alemu, and G Taxler (2016), “Tracking the diffusion of crop varieties using DNA fingerprinting,” Working paper, Ethiopian Institute of Agricultural Research (EIAR).