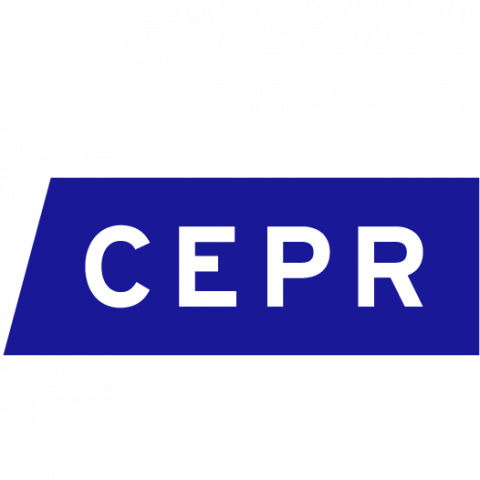
Revoking access to microcredit was costly – and not just for the households that borrowed
Microfinance is big business in India: in 2017, an estimated 40 million Indian households received 63 million microcredit loans from the approximately 223 microfinance institutions (MFIs) operating there (Ray2017). Previous randomised controlled trials (RCTs) in various contexts have focused on how borrowers stand to benefit from loans, either by investing in a household business (Banerjee et al. 2018, 2017) or accelerating consumption of durable goods such as buying a new roof (Ben-Yishay et al. 2017). While it’s important to evaluate borrowers’ outcomes at the individual or household level, it’s equally important to understand how the widespread availability of microcredit – approximately 20% of Indian households participate – affects the economy at a regional or even national level. These so-called general equilibrium impacts to the economy – which encompass changes to outcomes such as wages, employment, and consumption – are the focus of our research paper (Breza and Kinnan 2018).
Context: Andhra Pradesh’s ordinance
In October 2010, the state government of Andhra Pradesh (AP) in India issued an emergency ordinance, bringing microfinance activities in the state to a complete halt. The ordinance was a response to media reports of MFIs’ alleged coercive practices, which led to public outcry and backlash. The contraction in the supply of microcredit, caused by the ordinance, sent shockwaves far beyond Andhra Pradesh. Affected microfinance institutions could not collect on outstanding loans in AP, and larger lenders were reluctant to lend to microfinance institutions, fearing similar actions by other states. Because the emergency ordinance came as a surprise, the microfinance crisis that followed serves as a natural experiment to measure the impacts of the loss of microfinance on rural households.
Our study
To focus on the effects of microloans per se, and not the other aspects of the AP ordinance (such as the de facto forgiveness of outstanding loans that households in AP had at the time of the ordinance), we look at households living in districts outside of AP. These households were affected indirectly, to the extent that the lenders operating in their districts were affected. Microlenders with a large presence in AP at the time of the ordinance experienced default on a large share of their total loan portfolios, and therefore cut back sharply on issuing new loans in the districts outside of AP where they operated. Other lenders, without exposure to AP, did not suffer any such wave of defaults, and were able to continue lending as usual (The Economic Times 2017).
The figure below shows how lending evolved over time. The dashed line shows lending by MFIs with low exposure to AP and the solid line shows lending by MFIs with high exposure to AP. Prior to October 2010, both groups of MFIs were experiencing similar rates of growth. After the ordinance was issued in October 2010, the lending patterns of the two groups diverged sharply. The low-exposure group continued to see growth in lending much as they did earlier, but the high-exposure group saw its lending fall sharply. This group of lenders did not begin to experience loan growth again until mid-2012. This is when the Reserve Bank of India assumed sole regulatory authority over the microcredit sector, resolving the risk of other states taking actions similar to that of AP.
This figure shows the intuition for our research strategy. We are able to compare outcomes in two types of districts: those where the lenders operating there before the ordinance were unexposed to the defaults in AP, versus those where the lenders were exposed to the AP defaults. In order for this contraction in lending to be a valid natural experiment, it needs to be the case that the exposed districts would have had similar rates of change in credit, wages, consumption, and so on but for the AP ordinance. The figure above shows that this is the case. Intuitively, the fact that microcredit loan products were highly standardised (in terms of lending criteria, loan size, interest rate, etc.) means that it does not matter whether a loan came from one MFI, or another.
RCTs and natural experiments
RCTs excel at creating ‘clean’ variation but typically do so on a small scale that cannot fully capture general equilibrium effects. On the other hand, occasionally a policy can create a natural experiment: a situation where, for reasons that are de facto random, only some parts of an economy are exposed to the change. If available, such natural experiments can encompass entire labour markets. RCTs and natural experiments have different insights to contribute to the ongoing evaluation of microfinance’s impact in developing economies. The two methods generate complementary knowledge that, taken together, paint a fuller picture of microcredit’s total impact.
Results: Reduced rural wages and disproportionate impact of the poor
It turns out, the loss of credit had a measurable impact on the economy. The lower credit supply was associated with a 5–9% drop in rural wages. Because people had less money to spend, consumer spending, investment, and entrepreneurship also dropped. These effects were experienced by everyone in the economy, regardless of whether they had directly received microfinance. The results show that revoking credit can be very costly.
Another insight from the study is that some households were disproportionately affected by the sudden loss of access to credit. Households in the poorest fifth of the income distribution, who rely on casual labour for a large share of their income, were worst affected by the fall in wages. Intermediate-wealth households, who are often best positioned to use a microloan to expand their business, experienced the largest declines in consumption.
Concluding remarks
One could argue that the short-term losses due to a reduction in credit borrowed could be offset by future gains from not having to make repayments on these loans. However, we hypothesise that the present losses would lead to volatility in wages, earnings, and consumption. Moreover, some effects of the credit crunch could persist, due to, for instance, durable investment or adjustment costs. Hence, the loss in total welfare resulting from a pronounced short-term consumption fall will not be fully offset by a future stream of slightly higher consumption.
Our results show that the actions of politicians in Andhra Pradesh had large negative externalities on microcredit supply to the rest of the country. Microfinance institutions were no longer able to finance creditworthy borrowers in other states, which in turn led to decreased wages, earnings and consumption. This episode shows that microfinance, despite its small loan sizes, can have meaningful impacts on rural economies.
References
Banerjee, A, E Breza, E Duflo and C Kinnan (2018), “Do credit constraints limit entrepreneurship? Heterogeneity in the returns to microfinance”, working paper.
Banerjee, A, E Duflo, and R Hornbeck (2017), “How much do existing borrowers value microfinance? Evidence from an experiment on building microcredit and insurance”, working paper.
Ben-Yishay, A, A Fraker, R Guiteras, G Palloni, N B Shah, S Shirrell, and P Wang (2017), “Microcredit and willingness to pay for environmental quality: Evidence from a randomised-controlled trial of finance for sanitation in rural Cambodia”, Journal of Environmental Economics and Management, 86:121–140.
Breza, E and C Kinnan (2018), “Measuring the equilibrium impacts of credit: Evidence from the Indian microfinance crisis”, working paper.
Ray, A (4 October 2017), “Microfinance institutions are struggling for survival. Here’s why”, The Economic Times.