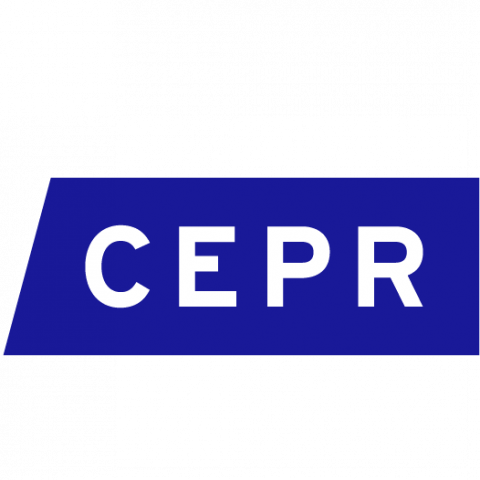
Optimising the assignment of tax collectors significantly increases tax revenue and compliance at little or no added cost
Governments have a key role to play in facilitating economic development, yet the public sector in low-income countries is often beset by inefficiencies (Finan et al. 2015). According to the World Bank’s Governance Indicators, low-income countries had an average percentile rank of 17.3 in government effectiveness in 2014, compared to 87.9 in high-income countries.
One way to tackle this problem is to improve how public-sector workers are assigned to teams and tasks. This approach is promising in light of recent evidence that the individual bureaucrats who make up the state explain a large share of the variation in government effectiveness (Best et al. 2019, Fenizia 2019). Moreover, a large body of evidence from the private sector suggests that team composition is an important determinant of worker productivity (Mas and Moretti 2009, Bandiera et al. 2010). Finally, governments are often unable to raise wages or promote workers based on their performance due to seniority-based civil service regulations. By contrast, improving worker assignment is a policy tool that is readily available to governments and, crucially, does not require additional revenue to implement.
In a recent working paper (Bergeron, Bessone, Kabeya, Tourek, and Weigel 2021), we investigate the optimal assignment of tax collectors in the Democratic Republic of Congo, a low-income state seeking to build rudimentary tax capacity. We study a property tax campaign in the city of Kananga in which tax collectors went door to door registering properties and collecting the annual property tax. Importantly, in each month of the campaign, 35 tax collectors were randomly assigned to new two-person teams, and these teams were randomly assigned to 184 neighbourhoods spanning 19,992 properties across the city. This two-staged random assignment allows us to study whether the tax authority could increase revenue collection by optimising the assignment of both collectors into teams and of teams to properties.
Defining the optimal assignment of tax collectors
The first step of our analysis is to define collector types (high and low). We do this first by splitting our sample into an analysis sample and a holdout sample. We then estimate collector type based on the tax compliance they realised in all neighbourhoods they were randomly assigned to in the holdout sample. Collectors were randomly assigned to 12 neighbourhoods each on average, so any differences in tax compliance attributable to specific neighbourhoods should balance out, providing us a with a measure of each agent’s effectiveness as a collector. We define high-type collectors as those who achieved tax compliance above the median level, and low-type collectors as those below the median.
The second step is to define household types (high and low). Here we rely on information from neighbourhood chiefs about local residents. Before tax collection, these chiefs predicted the ability of each household in the neighbourhood to pay the property tax. The chiefs’ estimates are highly predictive of subsequent compliance in the tax campaign, providing a convenient pre-campaign estimate of household type.
Having defined tax collector and household types, we estimate (in the analysis sample) the expected tax compliance across all combinations of collector-to-collector matches and collector team-to-households matches. We then search over all possible assignments on both of these dimensions to find the optimal assignment. This assignment maximises tax compliance while keeping collector teams’ workload and size the same as under the status quo assignment.
Stepping back, it is not obvious what kind of assignment would maximise tax compliance. If collection from high-propensity households is a straightforward task—akin to picking low-hanging fruit—then it could be optimal to assign them to low-ability collectors. Alternatively, if high-propensity households only pay if persuaded or visited when they have cash on hand, then the state may do better by assigning them to high-ability collectors. Similarly, in forming teams, one might expect that pairing one high-ability and one low-ability collector (mixed teams) would maximise compliance by ensuring that the essential tasks are all completed properly. However, there could also be scope for complementarity between collectors’ effort or skills that would justify grouping high-ability collectors together and low-ability collectors together (homogeneous teams). It is thus an empirical question what assignment will maximise tax compliance and revenue.
The optimal assignment matches high ability collectors together into teams—and high ability teams to high propensity households
We find that the optimal assignment involves positive assortative matching (Figure 1). To maximise tax revenue, the government should (i) form teams of exclusively high- or low-type collectors (no mixed teams), and (ii) assign high-type teams to high-type households and low-type teams to low-type households.
Figure 1 Optimal versus status-quo assignments
Note: This figure reports the optimal and the status-quo assignment of tax collectors to teammates and to households. Each bar represents the probability of each type of matching under the optimal (red) and status quo (blue) assignment. The first three entries on the x-axis show the assignment of tax collector pairs (low-low type or LL, high-low type or HL, high-high type or HH) to low-type households. The subsequent three entries on the x-axis show the assignment of collector pairs to high-type households.
The reason for such positive assortative matching is that the increase in compliance from adding a high-type collector to another high-type collector is considerably larger than the increase from adding a high-type collector to a low-type collector (Figure 2). In other words, there are complementarities in collector type.
We find evidence that these complementarities between high-type collectors arise because high-high collector teams appear to exert more effort, collecting for more total hours and on more distinct days than high-low or low-low collector teams. They also appear to direct their higher enforcement effort toward neighbourhoods with higher employment rates. This strategy of high-high teams—longer hours working in high employment neighbourhoods—likely boosts compliance because it increases the chances that property owners will have the cash on hand to pay the tax when the collectors solicit payment. The importance of liquidity constraints for property tax compliance resonates with recent evidence from Mexico (Brockmeyer et al. 2021).
Figure 2 Tax compliance by collector and household type
Note: This figure reports the average tax compliance when assigned to different types of collectors’ pair (low-low type or LL, high-low type or HL, high-high type or HL) by household type (low or high). The x-axis shows the three different types of collectors’ pair: LL, Hl, HH. The y-axis captures the tax compliance probability for different types of collector pairs and households. The coefficients for the high- and low-type households are shown in red and blue, respectively. The coloured lines show the 95% confidence intervals for each of the estimates.
Optimising the assignment of collectors to teams and tasks increases tax compliance by 37%
As in other low-income countries, property tax compliance in Kananga is low: 7.9% under the status quo assignment. According to our estimates, implementing the optimal assignment would increase compliance to 10.8% — a 37% increase (Figure 3). Tax revenue would also increase by 27%. The revenue gains would be about half as large if the government only optimised the assignment of collectors to teammates, or of collector teams to households. Each dimension of matching thus contributes roughly equally to the overall increase in compliance.
Figure 3 Estimated impacts of the optimal assignment on tax compliance
Note: This figure reports the impact of the optimal assignment policy on tax compliance in comparison to the status-quo (random) assignment. The first bar (in grey) reports tax compliance under the status-quo assignment. The second bar (in pale blue) indicates tax compliance when the government only optimises on the collector-to-collector dimension of the assignment. The third bar (in pale blue) reports tax compliance when the government only optimises on the collector-to-household dimension of the assignment. The fourth bar (in dark blue) reports tax compliance when the government implements the optimal assignment and optimises on the collector-to-collector and the collector-to-household dimensions of the assignment. The black lines show the 95% confidence intervals for each of the estimates.
One downside of the optimal policy is that, according to our analysis, it would cause a slight increase in household payment of collusive bribes to tax collectors in lieu of taxes. However, estimated bribe payments under the optimal assignment would remain low in frequency (2.18% of property owners) and magnitude (500 Congolese Francs, about 17% of the statutory property tax rate for most properties). We also find no evidence that the optimal policy would crowd out payment of other taxes or erode attitudes toward the government. Since high-type households tend to have more wealth and more valuable properties, the optimal policy would also increase the de facto progressivity of the tax.
Improving the assignment of tax collectors outperforms replacing collectors or increasing their wages
To benchmark the increase in tax compliance under the optimal assignment policy, we examine a series of alternative policies the government could implement.
First, we consider reassignment policies, in which the government reassigns neighbourhoods from low-type collectors to high-type collectors. To reach the same level of compliance as the optimal assignment policy, the government would need to reallocate 62% of neighbourhoods previously assigned to low-type collectors to high-type collectors (Figure 4, red line). Note that this estimate assumes that high-type collectors are just as effective at collecting taxes despite the increase in workload.
Figure 4 Effects of selection policies on tax compliance
Note: This figure shows the impact of the selection policies on tax compliance (y-axis). Selection policies involve reassigning a given percentage (x-axis) of the assignments that a low-type collector would receive under the status-quo assignment to other collectors. The red thick curve shows the estimated effects of the reallocation policy, where the workload is reassigned to existing high-type collectors in the sample. The blue thick curve shows the estimated effects of the hiring policy, where the workload is reassigned to newly hired collectors of average ability. The shaded areas represent 95% confidence intervals.
Second, we examine hiring policies, in which the government would reassign neighbourhoods to newly hired collectors of average collection ability. According to our estimates, this policy would never match the optimal matching policy, even if all low-type collectors were replaced (Figure 4, blue line).
Finally, we analyse whether the government could use performance-based financial incentives to motivate collectors to collect more tax revenue. To do this, we study random variation in piece-rate wages paid to collectors during the property tax campaign. The government would need to increase collectors’ performance incentives by 69% to increase tax compliance as much as the optimal matching policy. In practice, this policy would backfire — reducing net government revenue by 6% — because of the corresponding increase in collectors’ wage bills. The lackluster results from increasing collectors’ financial incentives underscore a key benefit of the optimal assignment policy: it is cost-neutral to implement.
Implications
Ultimately, the optimal assignment of tax collectors to teams and teams to neighbourhoods appears a promising way for governments in low-income settings to increase tax revenue without increasing the costs of tax administration. These results build on recent theory (Keen and Slemrod 2017) and evidence (Khan et al. 2015, 2018; Basri et al. 2021) that, in addition to investing in enforcement capacity (Besley and Persson 2009, Kleven et al. 2011, Pomeranz 2015), improving the efficiency of tax administration is paramount in low-income countries. Or, as Casanegra de Jantscher (1990) put it, “in developing countries, tax administration is tax policy.”
References
Bandiera, O, I Barankay and I Rasul (2010), “Social Incentives in the Workplace”, Review of Economic Studies 77(2): 417–458.
Bergeron, A, P Bessone, J K Kabeya, G Tourek, J L Weigel (2021), “Optimal assignment of bureaucrats: Evidence from randomly assigned tax collectors in the DRC”, Working Paper.
Besley, T and T Persson (2009), “The origins of state capacity: Property rights, taxation, and politics”, American Economic Review 99(4): 1218–44.
Basri, M C, M Felix, R Hanna and B A Olken (2021), “Tax Administration vs. Tax Rates: Evidence from Corporate Taxation in Indonesia”, Technical Report, National Bureau of Economic Research.
Best, M C, A Brockmeyer, H J Kleven, J Spinnewijn and M Waseem (2015), “Production versus revenue efficiency with limited tax capacity: Theory and evidence from Pakistan”, Journal of Political Economy 123(6): 1311-1355.
Brockmeyer, A, A Estefan, J C S Serrato, and K Ramirez (2021), “Taxing Property in Developing Countries: Theory and Evidence from Mexico,” Working Paper.
Casanegra de Jantscher, M (1990), “Administering the VAT”, in Gillis, M, Shoup, C S and Sicat, G P (Eds.), Value Added Taxation in Developing Countries, pp. 171-190, Washington DC, World Bank.
Fenizia, A (2019), “Managers and productivity in the public sector”, Technical Report, Mimeo.
Finan, F, B Olken and R Pande (2015), “The personnel economics of the state”, Technical Report, National Bureau of Economic Research.
Keen, M and J Slemrod (2017), “Optimal Tax Administration”, Journal of Public Economics 152: 133–142.
Khan, A Q, A I Khwaja and B A Olken (2016), “Tax farming redux: Experimental evidence on performance pay for tax collectors”, The Quarterly Journal of Economics 131(1): 219–271.
Khan, A Q, A I Khwaja and B A Olken (2019), “Making moves matter: Experimental evidence on incentivizing bureaucrats through performance-based postings”, American Economic Review 109(1): 237–70.
Kleven, H J, M B Knudsen, C T Kreiner, S Pedersen and E Saez (2011), “Unwilling or Unable to Cheat? Evidence From a Tax Audit Experiment in Denmark”, Econometrica 79(3): 651–692.
Mas, A and E Moretti (2009), “Peers at work”, American Economic Review 99(1): 112-45.
Pomeranz, D (2015), “No Taxation Without Information: Deterrence and Self-Enforcement in the Value Added Tax”, The American Economic Review 105(8): 2539–2569.