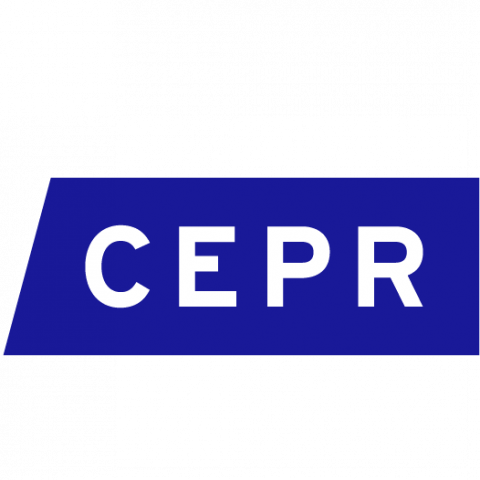
Governments can use satellites and machine learning technology to reduce illegal activity
Crucial modern technologies including cellphones and computers contain gold and other minerals which may have been sourced from illegal mines. For example, two-thirds of United States’ companies could not determine the origin of the minerals used in their supply chains (TGIATOC 2016). Illegal mining often occurs in resource rich areas that also have weak state capacity to regulate the industry, conditions that characterise many low- and middle-income countries in Africa, Asia, and Latin America, including Colombia. These impose significant costs on society – aside from evading taxes, illegal mines pollute the environment (Saavedra-Romero 2021), use child labor (ILO 2020), and finance armed groups (Rendón 2019).
One difficulty in controlling illegal activity is the government's inability to promptly measure its extent (Banerjee et al. 2012). In Colombia, it takes about two years to identify illegal mines using the manual inspection of satellite imagery (see Figure 1 for an example of this satellite imagery).
Figure 1: Example of a mine footprint observed from space
Source: https://comimo.sig-gis.com/
By contrast, the same inspection can be performed overnight using machine learning, which is the technology studied in Saavedra (2022) and used in Colombian Mining Monitoring (CoMiMo) (see Figure 2).
Figure 2: Example of mines detected with the monitoring technology
Source: https://comimo.sig-gis.com/
The intervention
I disclose the CoMiMo technology to the authorities, to address three research questions: (1) Does revealing the technology and location of illegal activity reduce the extent of the activity?; (2) Does the agent informed matter for the effect?; (3) Are there spillovers to neighboring areas not disclosed? I only disclosed five predicted mined locations per municipality; therefore, I can study spillovers to areas in treated municipalities that are not disclosed in the information letters. There might be positive spillovers (larger reductions in illegal mining) if the local authorities increase surveillance and reporting overall. But there might be negative spillovers if illegal activity relocates from disclosed locations to other areas.
I disclosed the technology and some mines identified by CoMiMo in a 2x2 Randomised Control Trail. In half of the municipalities, I informed local authorities (mayors) of the monitoring technology and five locations predicted as mines in their municipality. Note that I disclose the mining predictions without checking whether they are legal or illegal mines. For another random half of the municipalities, I informed the National Government (the Air Force) of five locations predicted as mined in each of those municipalities. That is, I randomly assigned municipalities to one of four groups: (1) information only to the local government; (2) information only to the National government; (3) information to both local and National governments, and (4) a control group, where I informed no agent.
Machine learning is prone to errors, and despite quickly making decisions on which areas are mined it is not 100% accurate. For an intuitive example see Figure 3 below, demonstrating how a Chihuahua can be confused with a blueberry muffin; in the case of mining, mines can be confused with a landslide or desert terrain. Consequently, when disclosing the technology and the predictions, I asked government authorities to verify if the model was correct. I compare what the authorities respond in the verification against double-blinded validation by students, which I consider as the truth. I find that local authorities are 16 percentage points less likely to confirm the model was correct when the mine is illegal versus a correct prediction of a legal mine. This differential accuracy is not present in the National authorities' responses. I take this differential response as evidence of collusion, illustrating the importance of independent monitoring technologies.
Figure 3: Machine learning technology is not 100% accurate
The effect of disclosing the technology on illegal mining
I study the effect of disclosing the technology on three different types of one-kilometer square grids: (i) β_D is the direct effect of what happens on a location disclosed in the information letter (denoted with a blue star in Figure 4), (ii) β_S captures what happens in the grids nearby a disclosed location, and (iii) β_T is what happens in grids in municipalities where I informed the authorities, but are farther than one kilometer from the disclosed points.
Figure 4: Illustrating spillovers
I find a reduction in illegal mining in the exact locations disclosed in the treatment letters (Figure 5, coefficients for “Disclosed” on the left). The treatment effects are similar regardless of which level of government is informed (blue: only local, red: only national, purple: both local and national informed). There is also a reduction of similar magnitude in surrounding areas less than 1km away from disclosed locations (Figure 5, coefficients for “Neighbour Disclosed” in the middle). However, there are also negative spillovers: an increase in illegal mining in areas of treated municipalities farther than 1km from disclosed locations (Figure 5, coefficients for “Muni treated” on the right). Without the negative spillovers, the reduction in illegal mining due to the information treatment would have been 11%, but still, even when accounting for increases in other areas which were not disclosed, the net reduction remains at 7%.
Figure 5: Effect of the intervention by grid type (spillover_unodc.pdf)
I then consider the mechanisms behind this effect, which shows that increased enforcement by government authorities drives the reduction in illegal mining. I find that treated municipalities started more sanctioning processes with National mining authorities and closed more illegal mines. Interestingly there is a reduction in illegal mining, even when the disclosed predictions for a particular municipality were inaccurate. This result illustrates that the threat of the monitoring technology is valuable, in addition to the information generated by the technology itself.
Finally, I investigate whether the information treatment affected other socio-economic and environmental variables. I do not find statistically significant effects on homicides or deforestation in treated municipalities. Although one could expect a reduction of deforestation, in Colombia the main driver of deforestation is livestock. One concern is that reducing illegal mining might affect the local population that economically depended on mining, but I do not find statistically significant effects on poverty or child labour. This result is in line with a mayors' survey, where around 80% state that workers from a closed mine switch to other occupations or migrate.
Conclusion
Six out of ten of the most severe risks that the world will face in the next decade are environmental (WEF 2023). Most of the remaining natural resources are located in developing countries with low state capacity, but new technologies are reducing the monitoring cost and can help strengthen state capacity, thus improving environmental protection. Similar monitoring technologies exist for other environmental issues such as deforestation (Global Forest Watch, GFoW), methane leaks (United Nations Environment Programme, MARS), fires (Copernicus Atmosphere Monitoring Service, CAMS), and illegal fishing (Global Fishing Watch, GFiW). Making sure government authorities use these new technologies will be critical for environmental protection.
References
Banerjee, A, S Mullainathan, and R Hanna (2012), “Corruption”, The Handbook of Organizational Economics, Princeton University Press.
Rendón O P M (2019), “La minería ilegal deja más plata que la coca”, El Colombiano.
ILO, International Labor Organization (2020), “Mapping interventions addressing child labor and working conditions in artisanal mineral supply chains”.
Saavedra, S (2022), “Technology and State Capacity: Experimental Evidence from Illegal Mining in Colombia”, Working paper available at SSRN.
Saavedra, S, M Romero (2021), “Local incentives and national tax evasion: The response of illegal mining to a tax reform in Colombia”, European Economic Review 138: 103843.
TGIATOC, The Global Initiative against Transnational Organized Crime (2016), “Organized crime and illegally mined gold in Latin America”.
World Economic Forum (2023), “Global Risks Report 2023”, Available at https://www.weforum.org/reports/global-risks-report-2023/digest